Harnessing Machine Learning for Inventory Control
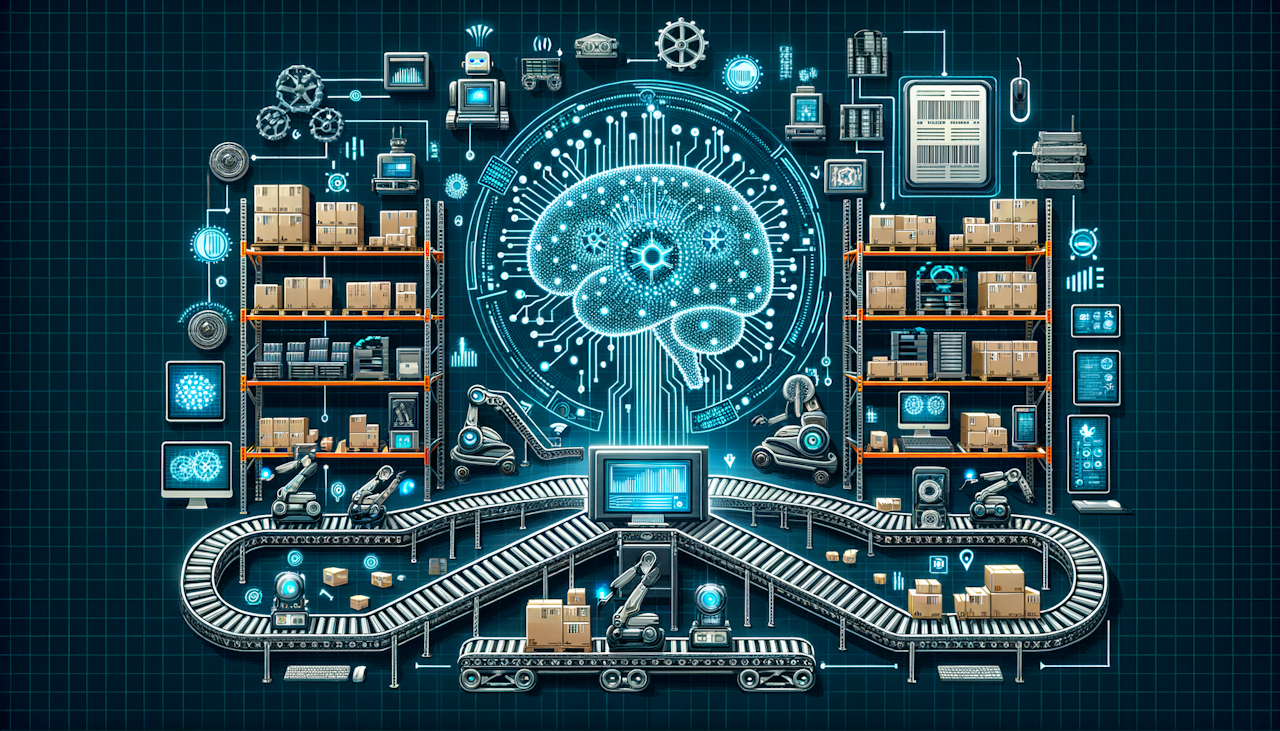
In recent years, technological advancements have significantly transformed the way companies operate, and an area ripe for innovation is inventory management. Machine learning, a subset of artificial intelligence, is at the forefront of this transformation, offering novel approaches to handling complex inventory systems.
The clairvoyance it bestows upon inventory managers allows businesses to anticipate and respond to market demands with unprecedented precision. This article probes the intersection of machine learning and inventory management, delineating why and how this cutting-edge technology is revolutionizing stock control, and ultimately, improving the overall efficacy of supply chain operations.
Understanding Inventory Management
Definition of inventory management
Inventory management is the orchestrated handling of stock and inventory to ensure that the right quantity of products is in the right place at the right time.
It is critical for maintaining the delicate balance between overstock, which could lead to increased holding costs, and understock, which can result in lost sales and customer dissatisfaction.
Importance of effective inventory management
Effective inventory management enables companies to minimize costs while boosting customer satisfaction. By efficiently overseeing stock levels, businesses can prevent revenue losses due to out-of-stock scenarios or excessive capital bound in unsold inventory.
This discipline is especially important for entities with large assortments of products or those operating in volatile markets.
Traditional methods of inventory management
Traditional inventory management often requires extensive manual effort, relying on heuristic rules and historical data for decision making.
Such approaches, while historically serviceable, are inflexible and cannot easily adapt to the rapidly changing market conditions and complex demand patterns observed in modern commerce.
Machine Learning: A Brief Overview
Definition and essence of machine learning
Machine learning is a branch of artificial intelligence that gives systems the ability to automatically learn and improve from experience. It involves algorithms that parse data, learn from that data, and then apply what they have learned to make informed decisions.
Different types of machine learning
Machine learning is broadly categorized into three types:
Supervised Learning: Where the system learns from a labeled dataset, providing answers based on the input-output mapping.
Unsupervised Learning: In this approach, the system is trained on an unlabeled dataset and is left to find structures and patterns on its own.
Reinforcement Learning: A method where an agent learns to behave in an environment by performing actions and noting the rewards or penalties.
Use cases and benefits of machine learning in various sectors
Beyond inventory management, machine learning has been successfully applied in areas such as healthcare for disease prediction, finance for fraud detection, and customer service for chatbots. The benefits include but are not limited to enhanced accuracy, process automation, and predictive capabilities.
The Role of Machine Learning in Inventory Management
Explanation of how machine learning works in inventory management
In inventory management, machine learning algorithms can analyze vast datasets to identify patterns that humans cannot.
It takes into account not only historical sales data but also other variables such as economic indicators, consumer trends, and even the weather to forecast demand more precisely.
Review of machine learning applications in inventory management
Machine learning significantly optimizes various aspects of inventory management:
Demand forecasting: Algorithmic trend analysis outperforms human foresight in predicting product demand, which is essential for inventory planning.
Production planning: Accurate demand predictions inform production schedules, ensuring that resources are allocated efficiently and without waste.
Warehousing and Distribution: Machine learning facilitates smarter warehousing solutions, optimizing everything from stock placement to the picking process, thereby reducing time and costs.
Illustration with suitable examples
A prime example of machine learning in inventory management is its ability to learn optimal stock levels across multiple locations, considering the transfer time and cost between these locations. This ensures the availability of products where and when they are needed while reducing the overall inventory holding costs.
The Benefits of Incorporating Machine Learning in Inventory Management
Enhanced forecasting accuracy
With access to complex predictive algorithms, businesses can achieve not only higher accuracy in their forecasts but also adapt to market trends more quickly, thereby staying competitive.
Improved operational efficiency
Automation of inventory-related tasks reduces the dependency on manual inputs and human errors while freeing staff to focus on more strategic responsibilities.
Reduced stock holding and shortage costs
A more nuanced understanding of demand patterns leads to the optimal stock levels, which ties up less capital in inventory and minimizes shortages that could otherwise result in missed sales opportunities.
Steps to Implementing Machine Learning in Inventory Management
Understanding the business requirements
Identifying the specific challenges and expectations of a particular business is the first step to successfully implement machine learning in inventory management.
Data collection and preparation
Machine learning models are only as good as the data they are fed. Collecting high-quality, relevant data plays a crucial role in the success of the model.
Choosing the right machine learning model
Businesses must select a model that aligns with their objectives, considering factors like the complexity of the data and the desired outcomes.
Potential pitfalls and challenges of using machine learning in inventory management
Lack of quality data
Not all companies have access to the extensive, clean data required to train effective machine learning models, which can hamper the implementation process.
Required technical expertise
Deploying machine learning solutions requires specialist skills that are currently in high demand, thus posing a challenge for many businesses.
High initial investment
Developing and introducing a machine learning-based system can be cost-prohibitive, especially for smaller enterprises that may lack the necessary capital.
Case Studies of Successful Implementation of Machine Learning in Inventory Management
Case Study 1: Retail sector (Preferred online shopping site)
An online retailer harnessed machine learning to optimize its inventory, resulting in a 10% reduction in warehouse costs and a 5% increase in customer satisfaction through improved stock availability.
Case Study 2: Manufacturing sector (Specific popular manufacturing company)
A global manufacturing firm implemented machine learning algorithms in its supply chain, enhancing its shipment forecasting accuracy by 20% and reducing rush orders by 30%.
Case Study 3: E-commerce sector (Specified online sales platform)
An e-commerce giant used predictive analytics to anticipate demand spikes, enabling it to stock products strategically and manage inventory turnover more efficiently.
Recap of the potential of machine learning in reinventing inventory management
The fusion of machine learning and inventory management presents an exciting frontier with the potential to transform how businesses approach stock control, demand forecasting, and supply chain efficiency.
Encouragement for businesses to adopt this technology and stay competitive in the market.
As industries continue to evolve and the digital marketplace expands, the adoption of machine learning in inventory management is not just a technological advancement but a strategic imperative for businesses intent on maintaining their competitive edge. As such, professionals seeking advancement in this area would benefit considerably from an online logistics certificate course or other relevant online courses with certificates that sharpen their understanding and skills in this high-demand domain.

Yu Payne is an American professional who believes in personal growth. After studying The Art & Science of Transformational from Erickson College, she continuously seeks out new trainings to improve herself. She has been producing content for the IIENSTITU Blog since 2021. Her work has been featured on various platforms, including but not limited to: ThriveGlobal, TinyBuddha, and Addicted2Success. Yu aspires to help others reach their full potential and live their best lives.