Time Series Analysis: Exploring Its Vital Role in Data Interpretation
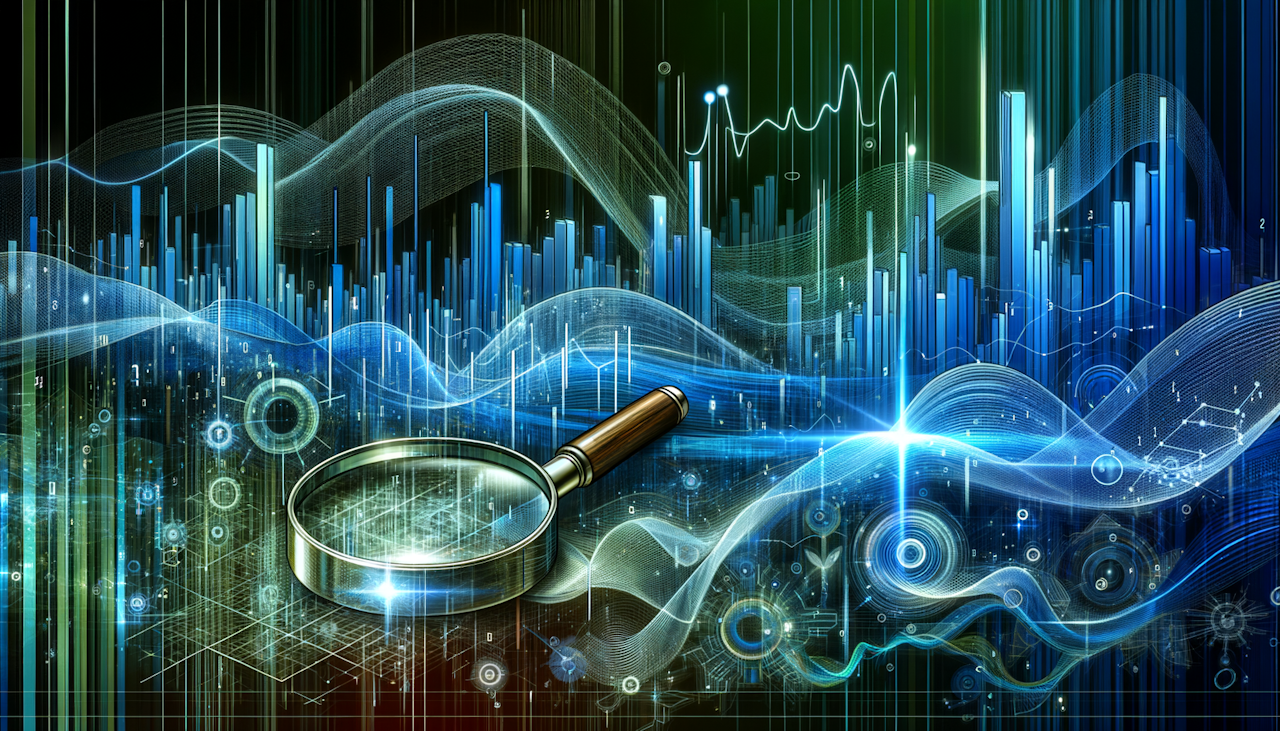
In the realm of data science and statistical analysis, time series analysis stands as a pivotal method for making sense of sequentially indexed data points. Such series are omnipresent, affecting diverse sectors from finance to meteorology. By examining how values change over intervals of time, we foster a richer understanding of underlying trends, cycles, and seasonal variations.
The predictive power of time series analysis reveals its indispensable role in decision-making processes and strategic planning. In this exposition, we explore the multiple facets of time series analysis, from its foundational principles to its complex applications, ensuring a comprehensive grasp of this vital analytical tool.
Understanding Time Series
Definition of Time Series
A time series is a sequence of numerical data points in successive order, typically measured at regular intervals. This can range from daily stock market prices to yearly rainfall measurements. The defining characteristic of a time series is that time acts as the independent variable, providing a frame to identify and measure changes and developments over duration.
Components of a Time Series
Trend Component
In time series data, the trend represents the long-term progression of the data, showing an overall direction in which the values are moving. Spotting a trend can often reveal a persistently increasing or decreasing behavior within the dataset, providing insight into growth or decline.
Seasonal Component
Seasonality refers to the recurring fluctuations that occur at regular intervals due to seasonal factors. For instance, retail sales typically spike during the holiday season, and this periodic increase is an important seasonality aspect to consider when analyzing sales data over time.
Cyclical Component
Cyclical components emerge as oscillations that do not have a fixed period. These undulating movements are attributable to economic conditions, business cycles, or other broad factors that influence the time series at inconsistent intervals.
Irregular Component
Lastly, the irregular component encompasses the random, unpredictable fluctuations which are neither seasonal nor cyclic. These can result from unexpected events or anomalies in the data, and often present a challenge during analysis.
Different Types of Time Series
Univariate Time Series
A univariate time series consists of single-variable observations at equally spaced time intervals. With just one variable to consider, these analyses can offer clarity and specificity, making it easier to identify patterns and predict future data points within that singular dimension.
Multivariate Time Series
Conversely, multivariate time series encompass observations of two or more variables. Capturing the complex interaction between these variables over time can provide more comprehensive insights but requires robust computational methods to decipher the intertwined effects.
Conceptual Framework for Time Series Analysis
Theoretical Assumptions
The framework of time series analysis is rooted in several theoretical assumptions. These presuppositions ensure the reliability and validity of the analytical conclusions. For example, it is generally assumed that the underlying mechanisms generating the time series are consistent over time, allowing past behaviors to project future values predictively.
Mathematical Concepts
Autocorrelation
Autocorrelation, or serial correlation, gauges the degree of similarity between a time series and a lagged version of itself over successive time intervals. High autocorrelation may indicate that past values have a significant influence on future values within the series.
Stationarity
A stationary time series is one whose statistical properties, such as mean, variance, and autocorrelation, are constant over time. For many analytical models, stationarity is a prerequisite, as it simplifies the structure of the series and the associated predictions.
Seasonality
Seasonality, as previously mentioned, consists of patterns that recur at consistent intervals due to seasonal factors. Accounting for seasonality in models is crucial to avoid mistaking these patterns for long-term trends.
Steps in Time Series Analysis
Data Collection and Pre-processing
Data acquisition and its subsequent refinement are critical initial steps in time series analysis. Proper collection methods ensure high-quality data, while preprocessing tasks like detrending and deseasonalizing can help to stabilize variance and mean, thus facilitating better model fit.
Model Selection
Autoregressive Models
Autoregressive models leverage the dependency between an observation and a number of lagged observations. These models are paramount when past values contain information that can be used to predict future values.
Moving Average Models
Moving average models are used to smooth out short-term fluctuations and highlight longer-term trends or cycles. They are particularly useful when the aim is not to predict future values but to understand the underlying trend or cycle.
Autoregressive Integrated Moving Average Models
These models, known as ARIMA, combine both autoregressive and moving average aspects but also include differencing of data points to achieve stationarity. They are versatile and widely applied in time series forecasting.
Model Fitting
Once the data is preprocessed and a model is selected, fitting the model involves estimating the parameters that best capture the dynamics observed in the data. This typically involves computational algorithms that minimize the error between the model's predictions and the actual data.
Diagnostic Checking
Diagnostic checks are integral in validating the adequacy of the model. Residuals (i.e., discrepancies between model outputs and true values) are analyzed for any remaining patterns that the model failed to capture.
Forecasting
The culmination of time series analysis is often to anticipate future values. A successfully validated model can be utilized to generate forecasts. The forecast's accuracy is paramount for strategic planning and decision making.
Practical Examples of Time Series Analysis
Time Series Analysis in Finance
Stock Market Analysis
The volatile nature of the stock market makes it a prime candidate for time series analysis. By examining past fluctuations in stock prices, analysts aim to forecast future price movements and guide investment strategies.
Economic Forecasting
Macroeconomic indicators like GDP, inflation rates, and employment figures are often analyzed as time series. Understanding the trend and cyclical components of these series aids governments and businesses in planning for future economic conditions.
Time Series Analysis in Healthcare
Disease Spread and Control
Time series analysis is instrumental in tracking the spread of diseases and evaluating the effectiveness of control measures. Predictive modeling can inform public health policies and responses.
Patient Monitoring
Continuous monitoring of patient vitals generates time series data that can be analyzed to detect abnormalities or predict health deteriorations, allowing for timely interventions.
Time Series Analysis in Weather Forecasting
Climate Change Studies
Long-term time series of climate data are crucial for studying the progression of climate change. Analyzing these series helps in the understanding and prediction of future climate conditions.
Seasonality Analysis
Weather phenomena exhibit strong seasonal trends, such as monsoon periods or hurricane seasons. Accurately modeling these patterns is essential for preparedness and risk management.
Advancements and Challenges in Time Series Analysis
Latest Developments in Time Series Analysis Techniques
Innovation in computational power and algorithmic design has led to advanced techniques in time series analysis. These developments have improved accuracy and expanded the complexity of series that can be analyzed.
Challenges in Implementing Time Series Analysis
Despite advances, there remain significant challenges such as dealing with high-dimensional data, structuring models for real-time analysis, and managing the inherent uncertainty in predictions.
Future Direction and Potential of Time Series Analysis
The field continues to evolve, promising enhanced predictive capabilities and insights. The future of time series analysis points towards greater integration of machine learning and artificial intelligence, potentially revolutionizing the way we interpret time-indexed data.

He is a content producer who specializes in blog content. He has a master's degree in business administration and he lives in the Netherlands.