Scatter Plot: A Comprehensive Guide to Visual Data Analysis
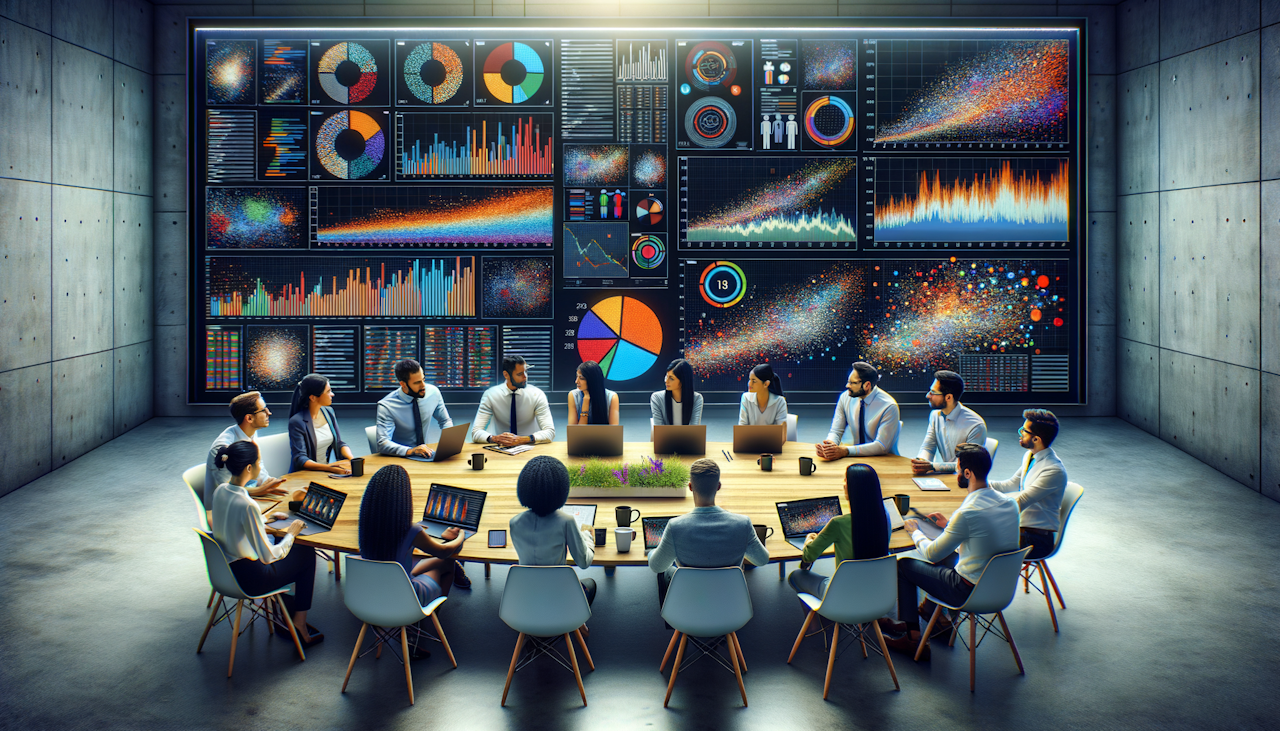
In the realm of data analysis, the visualization of complex information sets is crucial for the extraction of meaningful patterns and actionable insights. Within this context, the scatter plot emerges as an invaluable tool for researchers, statisticians, and business analysts alike. By plotting individual points on a graph, a scatter plot vividly illustrates the relationships between two variables, allowing analysts to surmise correlations and trends from seemingly disparate data points.
The importance of scatter plots cannot be understated, as they form the foundation upon which hypotheses are built and verified in the world of big data, statistics, and scientific research.
Understanding the Basics of Scatter Plots
Explanation of what Scatter Plots illustrate: Scatter plots fundamentally display the association between two variables by plotting data points on a two-dimensional graph. Each point on the scatter plot corresponds to an individual data record, with the position determined by the values of the two variables. Frequently used in exploratory data analysis, these plots are instrumental in discerning whether a relationship exists, and if so, the nature of that relationship—be it positive, negative, or non-linear.
Different components of a Scatter Plot: Fundamentally, a scatter plot is composed of two axes representing the variables of interest, with points plotted according to their value pairs. The horizontal axis, or x-axis, typically displays the independent variable, whereas the vertical axis, or y-axis, showcases the dependent variable. The scale of each axis must be considered carefully to accurately reflect the spread of data points. Additionally, scatter plots may include a line of best fit that suggests the central tendency of data points, providing a clear overview of any potential linear relationship.
How to read and interpret Scatter Plots: Interpreting scatter plots involves scrutinizing the distribution and pattern of the plotted points. A tight cluster of points suggest a strong relationship between variables, while a more scattered distribution indicates a weak relationship. The slope of the points, moving from left to right, indicates the direction of this relationship. A positive slope implies that as one variable increases, the other variable also tends to increase, whereas a negative slope suggests an inverse relationship.
Detailed Guide on How to Create Scatter Plots
Step-by-step process of creating Scatter Plots manually: Creating a scatter plot by hand involves the careful plotting of data pair values on a pre-drawn axis system. The initial step is to mark the values on each axis corresponding to the data set. Subsequently, each pair of values is represented as a point on the graph. Although constructing scatter plots manually provides fundamental insights into the plotting process, for large datasets, this approach becomes impractical, prompting the use of digital tools.
Utilizing software tools for creating Scatter Plots: In the modern digital era, numerous software tools offer the ability to generate scatter plots with efficiency and precision. Programs such as Excel or Google Sheets are equipped with functionalities that allow users to create these plots through easy-to-follow steps.
Specific tutorial on creating Scatter Plots using Excel: To create a scatter plot in Excel, one would begin by organizing data into columns, select the appropriate data range, and then navigate to the 'Insert' tab to find and select the 'Scatter' chart type. Further customization can be done to enhance readability and convey more information, such as adding trendlines or customizing the design of data points.
Specific tutorial on creating Scatter Plots using Google Sheets: Similar to Excel, Google Sheets provides a straightforward mechanism for crafting scatter plots. The process involves highlighting the dataset before accessing the 'Chart' option within the 'Insert' menu. Users can then customize their chart within the Chart Editor on the right, choosing the scatter plot option and adjusting the appearance as needed.
Real-world example of creating a complex Scatter Plot: Consider a scenario where a fitness center wants to analyze the correlation between time spent in the gym and weight loss among its members. By collecting data on hours spent exercising and the corresponding weight changes, analysts can use a scatter plot to visualize this relationship, potentially revealing insights into exercise efficiency and informing personalized training regimens.
Advanced Scatter Plot Concepts
Explanation and use of Scatter Plots with multiple data sets: Scatter plots can be extended to encompass multiple datasets, offering a kaleidoscopic view of the relationships across several variables. By differentiating the data sets with various colors or marker styles, an advanced scatter plot can provide a comparative analysis within the same visual framework, allowing for a more nuanced interpretation of the data.
Understanding correlation through Scatter Plots: One of the primary uses of scatter plots is to gauge the strength and type of correlation between two variables. Correlation coefficients can be calculated to quantify these relationships, but the scatter plot provides an immediate visual representation. A clear positive or negative slope of the data points is indicative of a strong correlation, while a more formless spread suggests little to no linear correlation.
Recognizing patterns and outliers in Scatter Plots: Beyond the primary trend, scatter plots are instrumental in identifying patterns, such as clusters or gaps, which can herald subgroups within the data or influential variables not accounted for. Furthermore, outliers, or data points that significantly deviate from the main cluster of points, can be easily spotted. These outliers could signal errors in data collection or represent anomalies that warrant further investigation.
Common Pitfalls and Misinterpretations in Using Scatter Plots
Discussion on common errors in creating and interpreting Scatter Plots: Errors in creating scatter plots often stem from mislabeling axes, choosing inappropriate scales, or neglecting to verify the integrity of the data before plotting. When interpreting scatter plots, common mistakes include assuming causation from correlation, overlooking the impact of outliers, and not recognizing the limitations of a scatter plot when it comes to complex, non-linear relationships.
Tips for avoiding these pitfalls: To evade these pitfalls, it's imperative to meticulously check the data before plotting, choose scales that accurately reflect the data distribution and explicitly label axes with units of measurement. When interpreting scatter plots, always approach with a critical mind, considering alternative explanations for observed patterns and seeking corroborating evidence before drawing conclusions.
Real-world examples of Scatter Plot misinterpretations: Real-life misinterpretations of scatter plots can have significant consequences. For instance, a public health researcher might erroneously conclude that an increase in ice cream sales leads to a rise in drowning incidents. Without considering the lurking variable—temperature and seasonal trends—the scatter plot alone might misguide the researcher away from the true underlying causative factors.
Conclusion
Scatter plots provide an informative glance into the intricate dance of variables within a dataset. Their ability to demystify complex relationships through a simple graphical representation renders them indispensable in the analytical toolkit. This guide has underscored not only the mechanics of creating and interpreting scatter plots but also their nuanced application in real-world scenarios. With an astute approach and a willing engagement with the underlying principles, users can harness the full potential of scatter plots in their analytic endeavors.

He is a content producer who specializes in blog content. He has a master's degree in business administration and he lives in the Netherlands.