Outlier Analysis: A Crucial Component in Data Interpretation
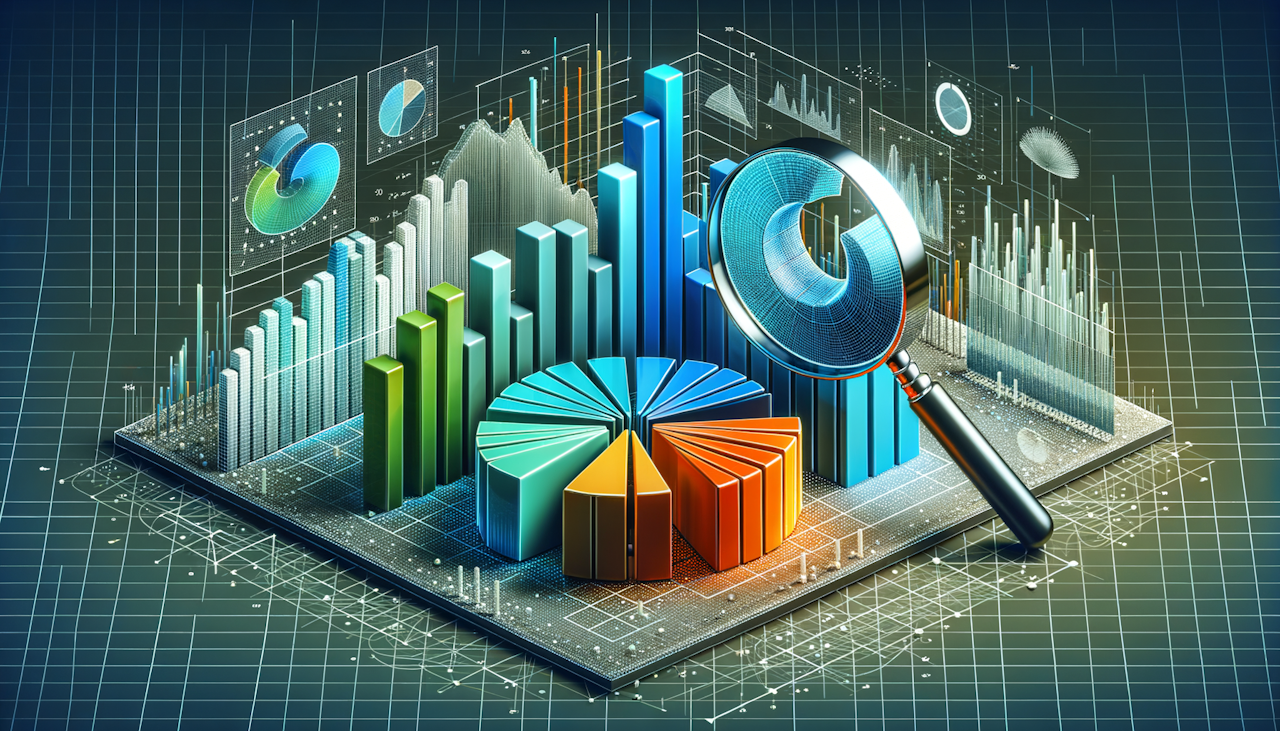
Outlier Analysis is the process of identifying and reacting to anomalous data within a dataset. These outliers can significantly skew results, leading to distorted conclusions and misleading information. As such, outlier analysis is a critical step in data interpretation and forms the crux of robust data analysis in various fields such as finance, healthcare, and cybersecurity. In this expansive blog post, we will delve deep into understanding outliers, explore the methods used to detect them, discuss their impact, and scrutinize how they can be effectively managed.
Furthermore, we will illustrate the real-world application of outlier analysis across industries, thereby emphasizing its fundamental role in data-driven decision-making.
Understanding Outliers
Explanation of what outliers are
An outlier is an observation that diverges dramatically from the norm within a dataset. From a statistical perspective, these are data points that fall outside of the overall pattern of distribution. Outliers can either be extremely high or extraordinarily low comparative to the rest of the data. Hence, identifying these unusual observations is essential for accurate analysis.
From a real-world perspective, outliers may represent innovation, error, or an underlying shift in a process. Understanding their nature is the first step in leveraging their presence for informed decision-making.
Causes of outliers
The emergence of outliers can typically be attributed to several causes. Data entry errors, such as mistyping or misreading values, introduce inaccuracies that can create outliers. Measurement errors occur due to faulty equipment or observer error, distorting the data. Experimental errors may arise from issues with the research design or execution.
And at times, intentional outliers are created through deliberate manipulation or fraudulent activity. Recognizing the sources of outliers is essential for determining the correct approach to managing them.
Types of outliers
Outliers come in various forms and understanding these can greatly aid in their identification. Univariate outliers stand out in one dimension, for example, an unusually high salary in a dataset of incomes. Multivariate outliers are atypical in several dimensions simultaneously – perhaps a data point with an unlikely combination of high income and low education in a socioeconomic dataset.
Global outliers are anomalous compared to the entire dataset, whereas contextual outliers are unusual only within a specific context or subset. Lastly, collective outliers could indicate a significant shift in data trends and would be critical to identify, as they may signal fundamental changes in behaviors or systems.
Methods of Detecting Outliers
Using Graphical Methods
Visualizations are potent tools for identifying outliers. A Box Plot, for instance, illustrates the distribution of data and clearly outlines data points that fall far outside the interquartile range. Scatter Plots can reveal outliers as points that deviate markedly from the data cloud. Furthermore, Z-Score plots can be used to visualize how many standard deviations a data point is from the mean; data points with a high Z-score are potential outliers.
Using Statistical Tests
A more empirical approach to detecting outliers is through statistical tests. The Z-Score Method is commonly used and identifies outliers by considering the number of standard deviations a datum is from the mean. Grubbs' Test is another test, suitable for detecting a single outlier by comparing the extreme values to the rest. Turkey's Test is akin to Grubbs’ but is better equipped for large data sets. However, these tests should be applied judiciously since they rely on assumptions about the data distribution that might not always hold true.
Using Machine Learning Techniques
Advanced methods for outlier detection incorporate machine learning algorithms. K-Means Clustering can detect outliers as points that do not belong to any cluster. DBSCAN, a density-based clustering method, identifies outliers as points in low-density regions. The Isolation Forest algorithm, on the other hand, isolates anomalies instead of profiling normal data points. When applying these machine learning techniques, practitioners should pay close attention to the hyperparameters and assumptions innate to each method as they significantly affect detection sensitivity and specificity.
Impact and Handling of Outliers
Explain the impact of outliers
Outliers can dramatically mislead data interpretation by distorting statistical measures like the mean and variance, leading to flawed analyses and decisions. Their impact on modelling and predictions is also noteworthy, as they can dramatically affect the performance and accuracy of predictive models. Being conscious of this impact is critical when dealing with outliers, as their presence can signify either noise that needs filtration or valuable data that may warrant further investigation.
Techniques of handling outliers
Several techniques are employed to handle outliers. Deletion is a straightforward approach but runs the risk of losing valuable information. Transformation methods, such as logarithms or square roots, can normalize data distributions, making outliers less pronounced. Imputation replaces outlier values with more typical values derived from other data points. Alternatively, one can opt to treat outliers separately, which can be a potent way of understanding anomalies without compromising the integrity of the dataset.
Evaluating the effects of these techniques
The critical takeaway when handling outliers is that the technique used should match the context and purpose of the analysis. When deciding which method to use, one should rigorously weigh the risks and benefits of each approach. Some techniques may introduce bias or change the structure of data. Thus, each method's effect on the interpretability and reliability of the results must be thoroughly evaluated to ensure that the final analysis continues to support sound decision-making.
Outlier Analysis in Practice
Use cases of outlier analysis across industries
Outlier analysis finds applications across diverse fields. In the finance sector, identifying outliers can aid in detecting fraudulent transactions. In the healthcare industry, outliers in patient data may indicate rare diseases or errors in recording information. Cybersecurity relies on outlier analysis to identify unusual network traffic that could signify a security breach. Supply chain management uses outlier detection to spot discrepancies in inventory or logistics data, which could indicate inefficiencies or errors in the workflow.
Example explanations showcasing the real-world impact of outliers and their handling
Consider the case of fraudulent credit card usage, an outlier in transaction patterns can alert analysts to possible criminal activity, potentially saving both financial institutions and customers from losses. In healthcare, outliers in patient recovery rates may uncover new treatment methods or care management practices that could enhance patient outcomes. The ability to handle these scenarios effectively, through robust outlier analysis, is essential for translating these insights into actionable intelligence.
Conclusion
In summary, outlier analysis serves a pivotal role in refining data accuracy and, by extension, the quality of the insights derived from it. We have outlined the various types of outliers, methods to detect them, and strategies for managing their impact. By appreciating the implications of outlier analysis and applying it judiciously across domains, organizations can sharpen their problem-solving skills training and support data-informed decisions. Further education on the subject, such as an online certificate course in data analysis, can empower professionals with the skills to navigate and capitalize on the intricacies of outlier analysis. In the age of big data, mastering outlier analysis is not merely an academic pursuit but a necessary skill for anyone looking to harness the full power of data in their respective fields.

He is a content producer who specializes in blog content. He has a master's degree in business administration and he lives in the Netherlands.