Multivariate Analysis: An In-depth Exploration in Academic Research
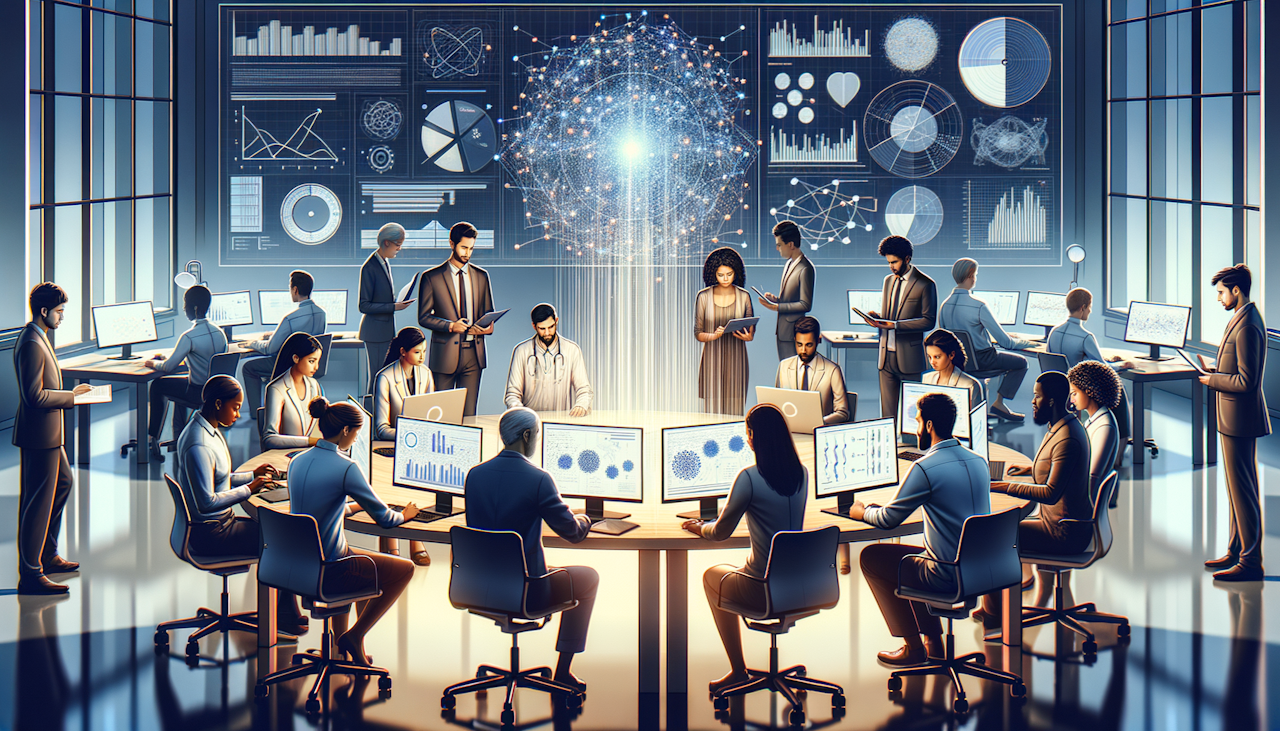
Multivariate Analysis encompasses a broad span of techniques used in the interpretation of datasets that include multiple variables or observations. This statistical landscape offers a nuanced perspective that stretches beyond the scope of univariate and bivariate analysis—which analyze one or two variables, respectively. In academic research, multivariate analysis stands as a critical tool, allowing researchers to understand complex phenomena and to tease out relationships between variables that may not be readily apparent.
An understanding of this method is invaluable, particularly as data becomes increasingly intricate and multifaceted. The correct application of multivariate analysis can lead to significant advancements in various fields, from psychology to biology, marketing, and beyond.
Understanding the Concept of Multivariate Analysis
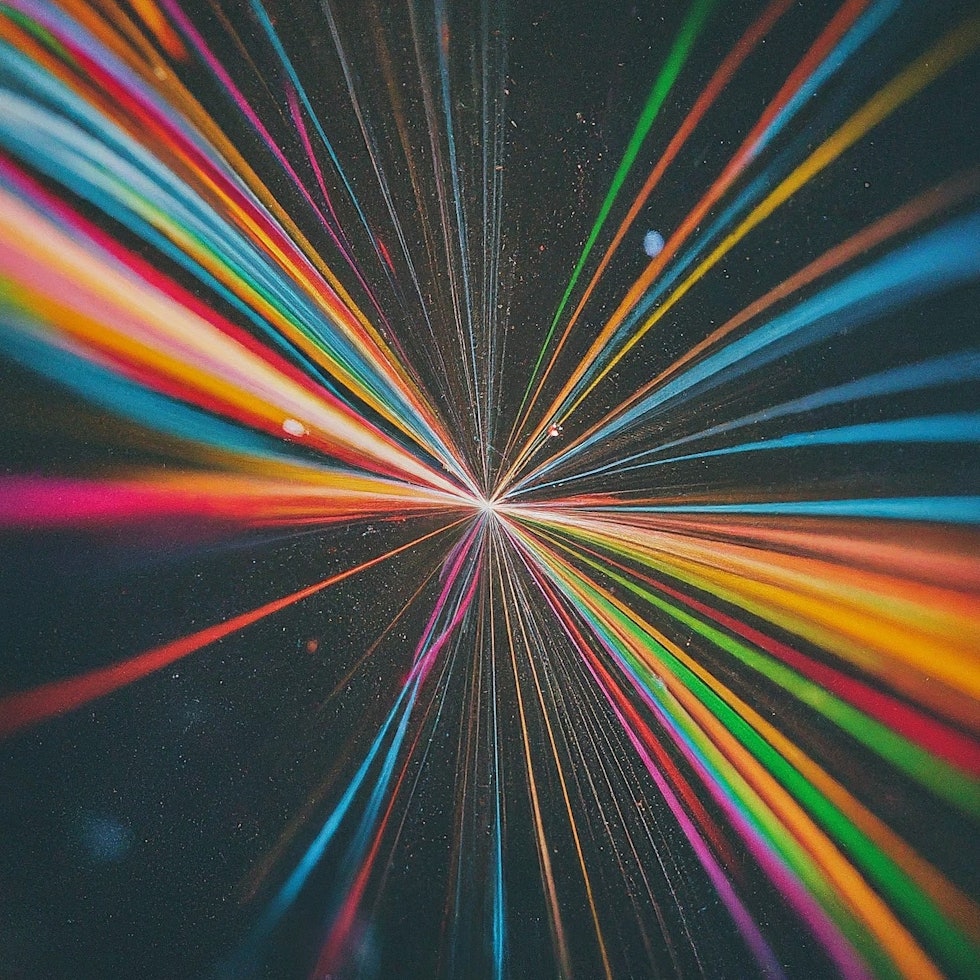
Detailed Explanation of Multivariate Analysis
Multivariate Analysis refers to any statistical procedure that simultaneously analyzes more than two variables on a set of data. It is a cornerstone of data analysis, predicated on the principle that multiple measurements, taken together, can provide a more comprehensive understanding of the phenomena under study. With its roots in quantitative research, this method facilitates the exploration of patterns, relationships, and differences within a dataset, managing the complexity inherent in multi-dimensional data structures.
Different Types of Data in Multivariate Analysis
In the application of multivariate analysis, researchers encounter various forms of data. These can be continuous measurements, such as weight or height, or categorical data, such as gender or species. The complexity arises when these different types of data interact within the same analytical framework, requiring robust, sophisticated methods to unpack the myriad of potential interactions and extract meaningful conclusions.
The Purpose of Using Multivariate Analysis in Research
The primary aim of using multivariate analysis in academic research is to understand the relationships between multiple variables and to determine the pattern and structure of these relationships. It is particularly valuable when variables are interdependent and the true pattern of these connections can only be elucidated through a simultaneous analysis, helping to control for the influence of multiple confounding factors. In a sense, multivariate analysis serves as the lens that brings the intricate web of data into focus.
Example of Using Multivariate Analysis in a Case Study
An educational psychologist may use multivariate analysis to examine the effects of socio-economic status, intelligence, and instructional methodology on students' academic performance. By considering these variables together, the researcher can assess their independent contributions to performance outcomes, as well as any interaction effects that occur when these factors converge.
Advantages and Disadvantages of Multivariate Analysis
The Benefits of Utilizing Multivariate Analysis
One of the most compelling advantages of multivariate analysis is its ability to handle the complexity and reality of many research settings where multiple variables are at play. It provides insights into data that would be impossible to detect by looking at variables in isolation. Additionally, by understanding the multivariate relationships, researchers can develop more accurate models for prediction and classification purposes.
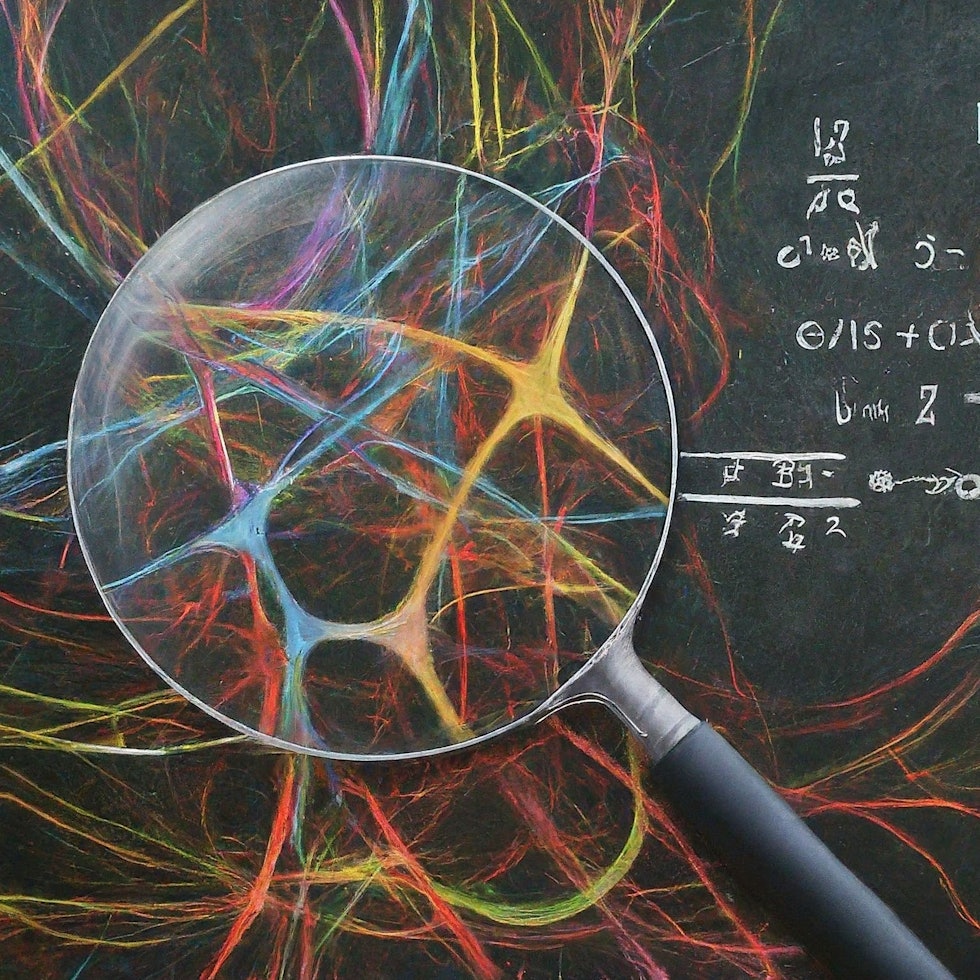
The Limitations and Challenges of Multivariate Analysis
However, multivariate analysis is not without challenges. It can be susceptible to overfitting, where models become too complex and perform well on the training dataset, but not on new data. Moreover, the interpretation of results can be complex and requires a deep understanding of statistical principles. Ensuring that the analysis is properly applied and its conclusions valid requires a high level of expertise.
Comparative Analysis: Multivariate against Univariate and Bivariate
Comparatively, multivariate analysis can illustrate a more complete picture than univariate and bivariate analyses, which look at single or pairs of variables, respectively. Multivariate analysis can reveal hidden structures and patterns that those simpler analyses cannot detect.
Providing Real-life Examples for Each Point Discussed
In marketing research, multivariate techniques such as cluster analysis can help identify different customer segments based on purchasing habits, age, income, and preferences, all considered simultaneously. In contrast, bivariate analysis might only reveal a simple relationship between two variables, such as income and spending, without considering the multifaceted nature of consumer behavior.
Essential Techniques of Multivariate Analysis
Introduction to Various Techniques
There are numerous techniques within the scope of multivariate analysis, each suited to different types of research questions and data structures. These include multiple regression analysis, factor analysis, cluster analysis, and more.
In-depth Look at Multiple Regression Analysis: Multiple regression analysis is a visionary technique for modeling the relationship between a dependent variable and several independent variables. It allows researchers to ascertain the extent to which each predictor variable explains the variation in the dependent variable, controlling for the effects of the other variables in the model.
Explanation of Multiple Regression Analysis: This method constructs an equation whereby the dependent variable is predicted based on a linear combination of the independent variables. It translates complex relationships into a solvable linear equation, enabling the exploration and prediction of the impact of changes in the predictors.
Application of Multiple Regression Analysis: For instance, in the context of problem solving training, multiple regression could be utilized to predict the success rate of students in a training program by considering a range of predictor variables, such as prior knowledge, training intensity, and individual motivation levels. This can provide valuable insights into how different factors contribute to the effectiveness of the training.
Detailed Discussion on Factor Analysis: Factor analysis is another robust multivariate technique used to identify the underlying construct or "factors" that explain the variations and covariances among observed variables.
Explanation of Factor Analysis: The essence of factor analysis lies in data reduction. It aims to simplify the complexity in datasets by reducing a large number of variables into a smaller set of latent factors without losing significant information.
Application of Factor Analysis: In psychology, factor analysis might be employed to discern key personality traits from a broad array of behavioral indicators, thus reducing the complexity of psychological constructs into principal components such as the renowned "Big Five" personality traits.
Examination of Cluster Analysis: Cluster analysis is geared towards grouping a set of objects in such a way that those within the same group (or cluster) are more similar to each other than to those in other groups.
Explanation of Cluster Analysis: While factor analysis reduces the variable space, cluster analysis organizes the observation space. It is a method of creating a taxonomy of multivariate data by sorting individuals or cases into homogenous and distinct groups.
Application of Cluster Analysis: An example of cluster analysis could be seen in biomedical research, where it helps to identify subgroups of patients based on multiple physiological variables, thereby contributing to targeted and personalized treatment plans.
Case Study Examples for Each Technique Discussed: Case studies across academia demonstrate the potency of these techniques. Online certificate programs on topics such as data science frequently incorporate these techniques, providing practical insights into their application in real-world scenarios.
Application of Multivariate Analysis in Various Fields
Usage of Multivariate Analysis in Business and Marketing
In the corporate sphere, multivariate analysis helps companies to segment their markets, forecast sales, and design products that cater to consumers' preferences and needs, informed by a combination of demographic, psychographic, and behavioral data.
The Role of Multivariate Analysis in Psychology and Social Sciences
In psychology and social sciences, multivariate analysis fosters a deeper understanding of human behavior and social patterns by analyzing multiple factors simultaneously. This could be crucial in fields like developmental psychology, where multiple aspects of human growth and changes over time are studied.
How Multivariate Analysis is Applied in Medical and Health Research
Medical and health research sectors benefit from multivariate techniques by examining the interplay between various risk factors and health outcomes. This approach is fundamental in building predictive models for diseases and in the assessment of treatment efficacy.
The Application of Multivariate Analysis in Other Relevant Sectors
Other sectors, such as environmental science or engineering, utilize multivariate methods to tackle complex issues ranging from climate change modeling to optimizing manufacturing processes.
Conclusion
Recap of Key Themes Discussed in the Article
This exploration of multivariate analysis has traversed its core concepts, advantages, limitations, and applications across a breadth of academic fields. We have elucidated the intricacies and impact of multivariate techniques that facilitate a compelling understanding of the patterns and structures within complex datasets.
Final Thoughts on the Impact and Importance of Multivariate Analysis in Research
The far-reaching implications and versatility of multivariate analysis affirm its central role in contemporary research paradigms. Despite its challenges, the precision and depth it brings to data interpretation are invaluable.
Encouragement for Further Study and Understanding
As we continually seek to unravel the intricacies of the world around us, a robust grasp of multivariate analysis is indispensable for any researcher. The burgeoning field of data science, punctuated by a plethora of online certificate programs, stands as a testament to the burgeoning demand for expertise in multivariate techniques. For those inclined toward empirical investigation, a solid foundation in multivariate analysis is not merely beneficial—it is essential.

He is a content producer who specializes in blog content. He has a master's degree in business administration and he lives in the Netherlands.