Monte Carlo Simulation: A Comprehensive Method for Risk Analysis
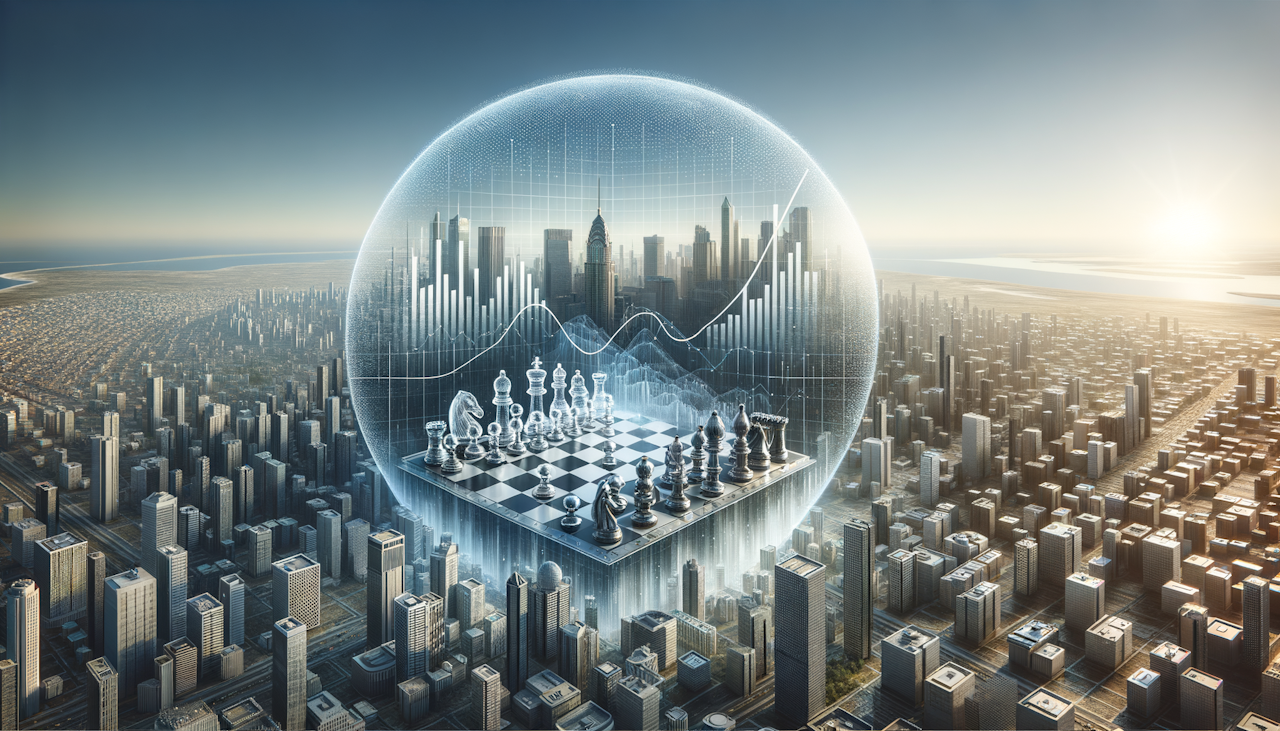
Introduction
In the current era of unpredictable market trends and complex project metrics, Monte Carlo Simulation stands out as a revolutionary technique capable of providing insight and foresight into the world of risk analysis and decision-making. This stochastic technique, which uses randomness to simulate the behavior of complex systems, has become an invaluable tool in various sectors, from finance to engineering. In this article, we will explore the depth and breadth of Monte Carlo Simulation, touching upon its rich historical nuances, fundamental concepts, and practical applications that underscore its importance across diverse fields. As industries continue to evolve and embrace technology, the role of sophisticated methodologies like Monte Carlo Simulation in navigating uncertainty has only amplified, further enhancing the critical nature of understanding its operations and benefits.
Historical Background of Monte Carlo Simulation
Genesis of Monte Carlo Simulation
The concept of Monte Carlo Simulation dates back to the 1940s when it was first employed by scientists working on the atomic bomb project at the Los Alamos National Laboratory. Named after the Monte Carlo Casino in Monaco, this simulation technique was used due to its reliance on chance and random events, reminiscent of gambling. The groundwork laid by the early applications of the Monte Carlo method set the stage for its incorporation into a wider array of domains, each benefiting from its capacity to simulate complex systems and predict future states under uncertainty.
Contributions of Pioneers
Key figures such as Stanislaw Ulam, John von Neumann, and Nicholas Metropolis contributed to the development and refinement of this methodology. Ulam inspired the approach by applying it to solve problems in mathematical physics, while von Neumann facilitated the computational implementation. It was Metropolis who coined the term "Monte Carlo," and together, these pioneers introduced a method that would later become a mainstay in probabilistic analysis and optimization problems.
Evolution Over Time
As computational power surged over the decades, the Monte Carlo Simulation underwent an evolutionary leap, expanding its capabilities and areas of application. No longer bound by the strict limitations of early computing resources, today's Monte Carlo techniques boast greater speed, efficiency, and accuracy, fueled by the technological advancements that have transformed megacomputers into compact, high-speed processors - thereby making the Monte Carlo method even more accessible and effective.
Understanding the Monte Carlo Method
Basic Definition and Concepts
The Monte Carlo method is a computational algorithm that relies on repeated random sampling to obtain numerical results, primarily used to approximate functions and simulate the behavior of complex systems. It is particularly effective in situations where traditional analytical methods are difficult or impossible to apply. The essence of the method lies in converting deterministic problems into probabilistic ones, thus allowing for a more manageable form of analysis.
Statistical Basis of Monte Carlo Method
Statistically speaking, the Monte Carlo method draws upon probability theory and inferential statistics. By simulating a vast number of trials, the method creates a distribution of possible outcomes that can be analyzed to gain insights into the likelihood of various scenarios. This is particularly beneficial when dealing with variables that have inherent uncertainty and are influenced by a multitude of random factors.
Steps Involved in Monte Carlo Simulation
A standard Monte Carlo Simulation involves several key steps: defining a domain of possible inputs, generating inputs randomly from a probability distribution over the domain, performing a deterministic computation on the inputs, and aggregating the results. Through this process, one can estimate quantities such as means, variances, and quantiles, effectively translating uncertain parameters into a range of potential outcomes.
Application Areas of Monte Carlo Simulation
Risk Assessment in the Financial Sector
Monte Carlo Simulation has found a vital role in the financial sector, where it is employed for valuing and assessing the risk of complex financial instruments, investment portfolios, and in the modeling of stock prices. By accounting for the volatility and random nature of markets, financial analysts can better prepare for a range of economic scenarios, balancing the risk versus reward of potential investments.
Usage in Project Management
Project managers leverage the power of Monte Carlo Simulation to predict the completion dates and budget requirements for large projects. By simulating the various factors that can affect a project timeline, from resource allocation to unforeseen delays, the method provides a probabilistic time frame and budget, thus aiding in more accurate planning and allocation of resources.
Relevance in Physics and Computational Biology
In fields such as physics and computational biology, Monte Carlo methods are crucial tools for studying systems with many coupled degrees of freedom, such as fluids, disordered materials, and biological pathways. Researchers utilize these simulations to predict molecular behavior, thermal fluctuations, and the cascading effects within large biological systems.
Benefits of Using Monte Carlo Simulation
Decision Making Assistance
The ability of Monte Carlo Simulation to present a range of possible outcomes and their respective probabilities provides a solid foundation for decision making under uncertainty. This is especially beneficial in strategic planning and investment decisions where the stakes are high and the variables, plentiful.
Uncertainty Analysis
Monte Carlo Simulation shines in uncertainty analysis by allowing modelers to readily identify the variables that impact the system the most. By observing how variations in these variables affect outcomes, businesses and researchers can better understand where to focus their attention and resources.
Complex Problem-Solving
The versatility of the Monte Carlo method makes it an excellent choice for solving complex problems that involve a significant number of variables and which cannot be solved by simple analytical methods. It is particularly adept at addressing problems within multidisciplinary fields that involve interconnected systems with dynamic variables.
Practical Examples of Monte Carlo Simulation
Case Study in Finance Sector
An example of Monte Carlo Simulation in finance can be observed in the valuation of options and derivatives. Traders use the method to forecast future price movements and volatility, thereby tailoring their investment strategies to hedge against potential losses.
Monte Carlo in Project Deadline Management
In project management, the simulation assists in creating a probability distribution for a project's completion date. This helps in identifying the likelihood of meeting deadlines and enables project managers to adjust schedules and resources accordingly.
Use in Logistic Regression Analysis
Monte Carlo Simulation is used in statistical analysis, such as logistic regression, to assess how well a model fits data when the underlying assumptions of traditional methods are not valid. This can be crucial when researching areas like medicine or social sciences, where the model's implications have direct real-world consequences.
Limitations and Challenges of Monte Carlo Simulation
Concerns Regarding Accuracy
While Monte Carlo Simulation can provide a wealth of information, its accuracy is highly dependent on the quality and quantity of the input data. Inadequate or incorrect data can lead to misleading results, and thus, the validity of the findings hinges on the initial inputs.
Computational Demands
The extensive computational power required to perform numerous iterations can be a limiting factor, especially for simulations of very complex systems or when fine resolution of uncertainty is required. Advances in computational hardware continue to mitigate this issue; however, it remains a consideration when employing the method.
Dependence on Quality of Input Data
The adage "garbage in, garbage out" is pertinent when discussing Monte Carlo Simulation. The output is only as reliable as the input data, and erroneous or biased inputs can significantly detract from the simulation's value. Ensuring high-quality data is therefore paramount to the effective application of this method.
Future of Monte Carlo Simulation
Incorporation of Machine Learning and Artificial Intelligence
The fusion of Monte Carlo Simulation with machine learning and artificial intelligence heralds a transformative future for risk analysis and predictive modeling. As these technologies evolve, so does the potential for more sophisticated, adaptive, and nuanced simulations that can learn from data in real-time and adjust their parameters accordingly.
Possible Improvements and Advancements
Continued improvements in Monte Carlo Simulation are likely to stem from the ongoing development in computational capabilities, algorithmic efficiencies, and the integration of alternative stochastic processes. These advancements promise to enhance the speed, accuracy, and applicability of Monte Carlo methods across even more fields, suggesting a bright and versatile future for this powerful analytical tool.
Conclusion
Summary of Key Points Discussed
Monte Carlo Simulation represents a nexus of probability, statistics, and computational performance. Its utility in predicting outcomes under uncertainty has rendered it indispensable in numerous sectors. The ability to dissect complex problems and forecast a spectrum of possibilities makes Monte Carlo an invaluable ally in the ever-expanding domain of risk analysis.
Final Thoughts and Reflections on Monte Carlo Simulation
As we consider the trajectory of Monte Carlo Simulation, it is clear that its continued evolution will be closely tied to technological advancement and the pursuit of greater knowledge across all disciplines. Its widespread application and the substantial benefits it offers in decision-making under uncertainty reinforce its position as a centerpiece in quantitative analysis.
References
Further exploration into the intricate world of Monte Carlo Simulation and its applications can be pursued through various academic papers, textbooks, and online resources. For those interested in deepening their practical skillset, an online certificate course in this area can be pursued. Additionally, for individuals seeking to sharpen their analytical prowess without financial barriers, a problem solving course free of charge can provide foundational knowledge and enhance their understanding of Monte Carlo methods. These educational avenues serve as both a testament to Monte Carlo's significance and a pathway for aspiring students and professionals to drive innovation in their respective fields.

He is a content producer who specializes in blog content. He has a master's degree in business administration and he lives in the Netherlands.