How NLP Can Help You Understand Your Students
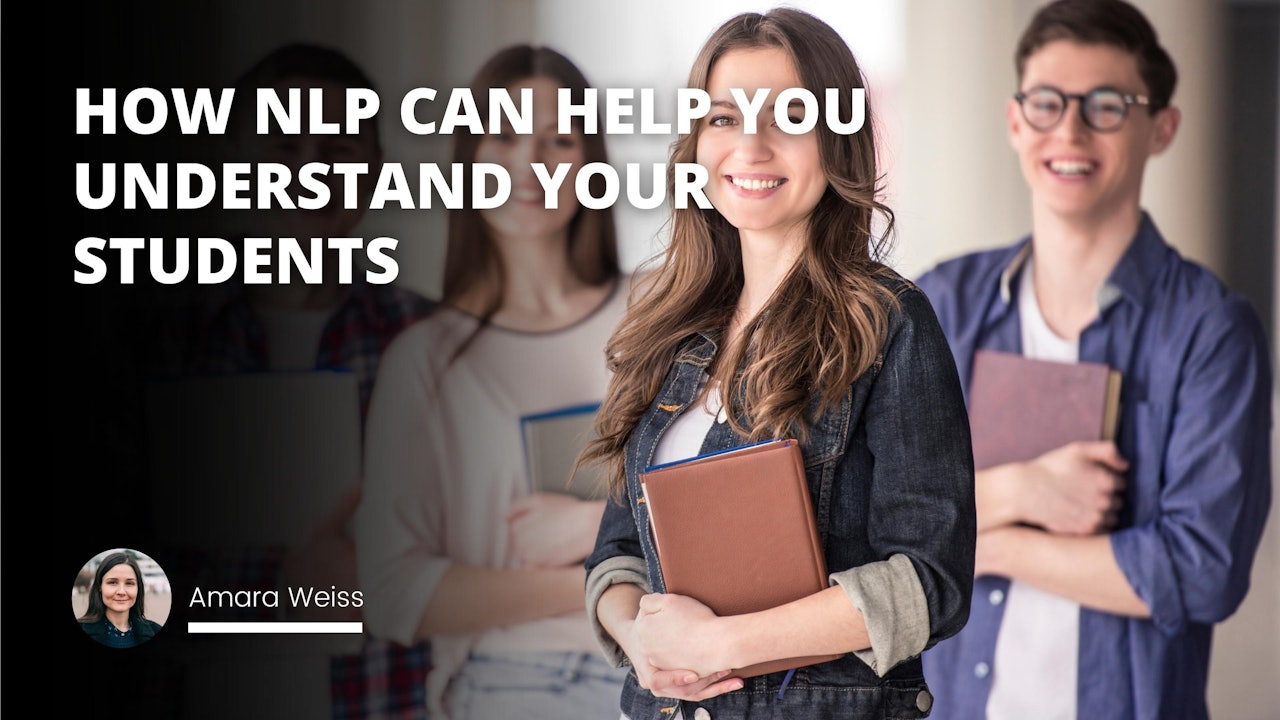
Aspect | Function | Benefit |
---|---|---|
NLP in Analyzing Student Writing Samples | Helps in determining student's proficiency level and identifying areas of improvement | Allows targeted academic support for individual students |
NLP in Processing Student Feedback | Gives a better understanding of student thoughts about the institution and its programs | Guides institution towards service improvement based on student's feedback |
NLP in Monitoring Social Media Activity | Identifies potential issues or concerns raised by students | Enables proactive measure to address issues and maintain good institution reputation |
NLP in Combination with Traditional Methods | Supplements conventional methods like surveys and interviews with a data-based approach | Provides comprehensive insights into student behavior and needs |
Text Classification via NLP | Sorts text data into organized categories based on linguistic patterns | Improves data analysis efficiency and accuracy |
Sentiment Analysis with NLP | Determines sentiments expressed in text to comprehend student's feelings | Helps in understanding student's attitudes towards learning and institution |
Topic Modeling using NLP | Extracts key themes or topics of discussion from text data | Identifies trending topics among students to develop relevant programs and initiatives |
NLP in Identifying Behavioral Patterns | Detects consistent behaviors among students | Informs and leads to early intervention in problematic behaviors |
NLP for Teachers | Empowers teachers to better understand and manage student communication and behavior | Reduces teacher stress and improves classroom management |
Case Studies | Offers practical instances showing NLP usage in identifying at-risk students, improving courses, and personalizing learning | Validates the effective implementation of NLP in educational institutions |
NLP can be used in several ways to understand students better. For example, NLP can be used to analyze student writing samples to determine their proficiency level or to identify areas where they may need additional help.
Additionally, NLP can process student feedback to understand better students' thoughts about the institution and its programs. Finally, NLP can also monitor student social media activity to identify potential issues or concerns. By using NLP, institutions can gain a wealth of insights into their students that would otherwise be difficult or impossible to obtain.
While NLP can be beneficial, it is essential to keep in mind that it is just one tool that can be used to understand students better. In many cases, combining different methods will produce the best results.
For example, using NLP to analyze student writing samples can be combined with traditional methods such as surveys or interviews to get a complete picture. Ultimately, the goal should be to use whatever tools are available to understand the students better so the institution can better serve their needs.
What is NLP, and how can institutions use it to understand their students better?
NLP, or Natural Language Processing, is a branch of artificial intelligence that deals with processing and understanding human language. NLP can be used for various tasks, such as text classification, sentiment analysis, and topic modeling.
Additionally, NLP can process student feedback to understand better students' thoughts about the institution and its programs. Finally, NLP can also monitor student social media activity to identify potential issues or concerns. By using NLP, institutions can gain a wealth of insights into their students that would otherwise be difficult or impossible to obtain.
While NLP can be beneficial, it is essential to keep in mind that it is just one tool that can be used to understand students better. In many cases, combining different methods will produce the best results.
For example, using NLP to analyze student writing samples can be combined with traditional methods such as surveys or interviews to get a complete picture. Ultimately, the goal should be to use whatever tools are available to understand the students better so the institution can better serve their needs.
NLP can be used in several ways to understand students better. For example, NLP can be used to analyze student writing samples to determine their proficiency level or to identify areas where they may need additional help.
Additionally, NLP can process student feedback to understand better students' thoughts about the institution and its programs. Finally, NLP can also monitor student social media activity to identify potential issues or concerns. By using NLP, institutions can gain a wealth of insights into their students that would otherwise be difficult or impossible to obtain.
How NLP can help identify patterns in student behavior
As any teacher knows, student behavior can be a challenge to manage. For example, some students are disruptive, while others seem unengaged or even hostile. Keeping track of all the different behaviors can be a daunting task.
However, new technologies are emerging that can help identify student behavior patterns. One such technology is called NLP, or natural language processing. NLP uses artificial intelligence to analyze text data, looking for ways that might otherwise be missed.
For example, NLP could identify students who are consistently late to class or frequently get into arguments with their peers. By identifying these patterns, teachers can take steps to address the underlying issues. As NLP becomes more sophisticated, it has the potential to revolutionize the way we understand and manage student behavior.
The benefits of using NLP to understand students better
Teachers have a lot on their plates. Between lesson planning, grading papers, and dealing with challenging students, it's no wonder they sometimes feel like they're in over their heads. However, there may be hope yet!
Neuro-linguistic programming, or NLP, is a field of study that explores the relationship between language and behavior. By better understanding how students communicate, teachers can more effectively manage their classrooms and help every student reach their potential. In addition, teachers who use NLP techniques report feeling less stressed and more confident in their abilities.
And who wouldn't want that? So the next time you're feeling overwhelmed by your job, remember that NLP could be the answer to all your problems.
Case studies of how institutions have used NLP to improve their understanding of students
Institutions have used NLP to improve their understanding of students' needs in various ways. For example, one school used NLP to identify at-risk students and offer them targeted support. Another used NLP to analyze student feedback and make course improvements.
And still, another used NLP to develop a more personalized approach to student advising. In each case, NLP proved a valuable tool for helping institutions better serve their students. As the use of NLP continues to grow, even more schools will likely find ways to use this powerful technology to improve the student experience.
Ways in which institutions can begin using NLP to understand their students better
If your institution is interested in using NLP to understand your students better, there are a few ways to get started. First, you'll need to gather data. This can be done through surveys, focus groups, or writing samples. Once you have this data, you'll need to process it using NLP techniques.
Finally, you'll need to interpret the results and take action based on what you've learned. By taking these steps, you can start using NLP to gain valuable insights into your students' needs and preferences.
Neuro-linguistic programming (NLP) is a field of study that explores the relationship between language and behavior. It can be used in several ways to understand students better so teachers can more effectively manage their classrooms. For example, NLP could be used to identify patterns in student behavior, such as those who are consistently late to class or frequently get into arguments with their peers.
Additionally, NLP could be used to analyze student feedback and make course improvements or to develop a more personalized approach to student advising. Ultimately, the use of NLP can revolutionize how we understand and manage student behavior.
Institutions that want to begin using NLP to understand their students better can start by gathering data through surveys, focus groups, or writing samples. This data can then be processed using NLP techniques to identify patterns and trends.
Finally, the results of the analysis should be interpreted and used to take action to improve the student experience. Institutions can use NLP to gain valuable insights into their student's needs and preferences by taking these steps.
References:
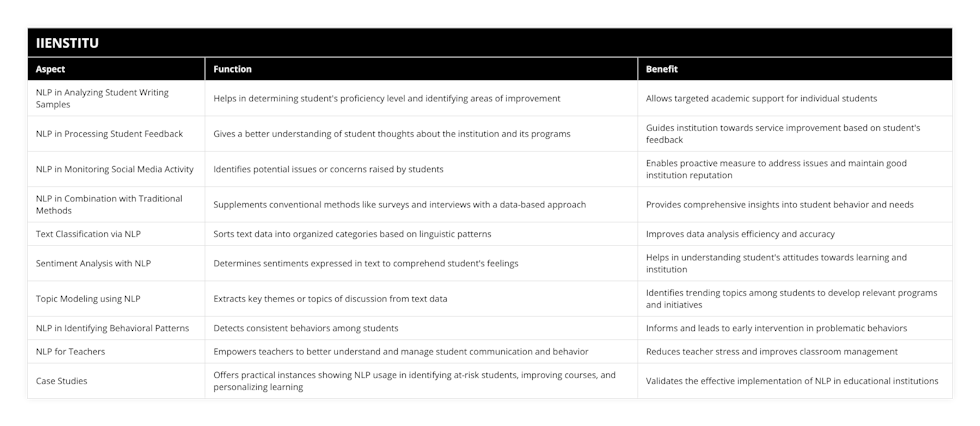

I am Amara Weiss and for many years I have worked in the field of education, specifically in the area of technology. I firmly believe that technology is a powerful tool that can help educators achieve their goals and improve student outcomes. That is why I currently work with IIENSTITU, an organization that supports more than 2 million students worldwide. In my role, I strive to contribute to its global growth and help educators make the most of available technologies.