Data Analysis: A Key Tool in Strategic Decision Making
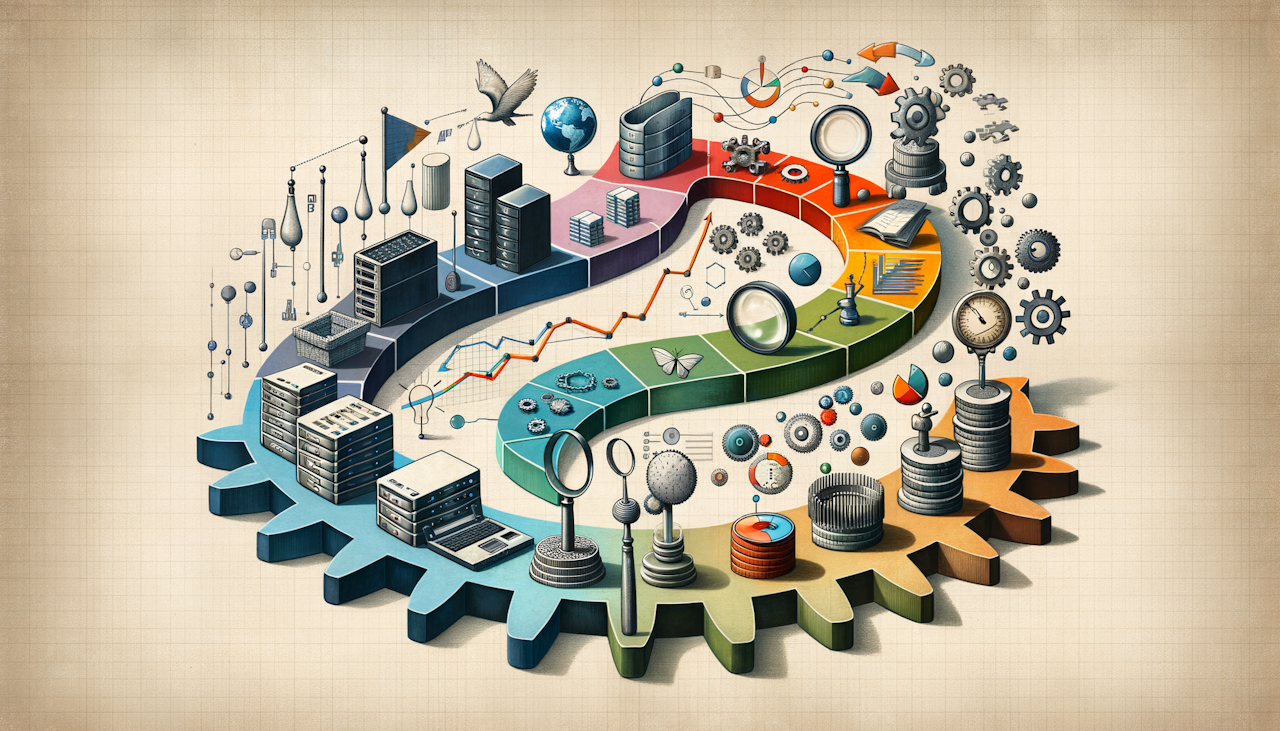
Data analysis has profoundly impacted strategic decision-making processes.
It empowers individuals and organizations by providing tools to inspect, cleanse, transform, and model data, leading to informed decision-making.
Descriptive Analysis summarizes historical data, while Diagnostic Analysis probes deeper to determine causation or correlations.
Predictive Analysis forecasts future events using historical data, while Prescriptive Analysis determines the best course of action in a given situation.
Advancements in data analysis include the emergence of AI and machine learning, allowing for real-time data processing and complex algorithmic predictions.
Data analysis stages include Data Collection, which is critical for the integrity of the analysis, and Data Cleaning, which involves identifying and correcting errors or omissions in the data.
The pervasive nature of data has fundamentally transfigured the dynamics of strategic decision-making in the contemporary era. Data analysis has emerged as a quintessential instrument in deciphering the intricate weave of digital information ubiquitous in all sectors of business and society at large. This blog endeavors to delineate data analysis's profound impact on strategic decision-making processes, underscored by an expert approach tilting towards statistics legend John W. Tukey's tone of voice.
Introduction to Data Analysis
As the cornerstone of informed decision-making, data analysis empowers individuals and organizations by providing the tools to meticulously inspect, cleanse, transform, and model data. This understanding allows us to discover helpful information, suggest conclusions, and support decision-making. In our increasingly data-driven world, the significance of data analysis cannot be overstated, as it fuels the knowledge that underpins innovation and efficiency across diverse industries. Within this article, we shall navigate the multifaceted landscape of data analysis, its types, stages, real-world applications, and a gaze into its promising future.
In this exploration, you'll gain insight into what constitutes proficient data handling and the importance of ethics, the avoidance of common follies, and the anticipation of future advancements. These dimensions offer a comprehensive narrative on deploying data for concrete actions and outcomes, fundamental to problem-solving skills training and online certificate courses designed to hone expertise in this domain.
Different Types of Data Analysis
Descriptive Analysis forms the bedrock of data analysis, painting a picture of 'what has happened' through historical data. It employs many statistical tools to summarize a dataset, be it through the mean scores of a student's academic performance, the median income of a population, or the mode of transport most used in a city. This kind of Analysis is typically the first step in data scrutiny, and it is potent in broadcasting a clear signal from the noise of raw data.
Diagnostic Analysis digs beneath the superficial to answer 'why something happened.' It takes the insights provided by Descriptive Analysis and probes deeper to discern causation or correlations. An example of this might be determining the reasons behind a sudden dip in sales following a month of exemplary performance. Organizations can rectify issues or capitalize on positive influencers by identifying the factors that contributed to this change.
Predictive Analysis harnesses historical data to forecast future events with a certain probability attached to its predictions. For instance, a retailer might use past sales trends to predict future customer behaviors and stock requirements. It is a complex form of Analysis that employs statistical models and machine learning techniques to make educated guesses about future possibilities.
Prescriptive Analysis is a forward-looking process that seeks to determine the best course of action in a given situation. Prescriptive analysts can suggest various outcomes and strategies for a business problem by using insights from all preceding forms of Analysis. For example, they can advise a company on the optimal marketing strategy for a new product launch by analyzing past campaign data and predicted market trends.
Advancements in Data Analysis
The ongoing evolution of data analysis techniques is partially powered by the emergence of cutting-edge technologies such as AI and machine learning, which have augmented our capabilities to process and interpret vast datasets. Modern analytics platforms can now efficiently handle real-time data processing and complex algorithmic predictions, fundamentally altering the data analysis landscape. Looking ahead, we can expect more sophisticated analytics tools and methodologies, driven by the acceleration in data volume, variety, and velocity. These future advancements will further enhance the role of data analysis in decision-making processes.
Artificial intelligence has revolutionized data analysis by enabling predictive models that self-improve over time. Machine learning algorithms, a subset of AI, can sift through enormous data sets to uncover patterns and insights invisible to human analysts, leading to more accurate and actionable intelligence for strategic decision-making. These technologies have augmented our capabilities to process and interpret vast datasets, leading to transformative changes in the field of data analysis.
Stages of Data Analysis
The inception of data analysis lies in Data Collection. The integrity of the Analysis hinges on the data quality, making this a mission-critical stage. From customer feedback forms to IoT sensors, the array of methods and sources for data collection is diverse and prosperous; however, the credibility and relevance of the data sources are paramount for reliable Analysis.
Data Cleaning is often described as the less glamorous but indispensable facet of data analysis. It involves identifying and correcting errors or omissions within data sets. The integrity and accuracy of data analysis are directly reliant on the thoroughness of this process, as even minor discrepancies can result in significant misinterpretations.
At the heart of the endeavor lies the actual Data Analysis. The clean data sets are subjected to various statistical and analytical processes to extract meaningful patterns, trends, and relationships. Analysts apply different lenses of analysis tools to the data, contingent on the objectives outlined at the onset.
Understanding the story behind the numbers is critical in Data Interpretation. Analysts use this process to make sense of the outcomes of statistical tests, constructing a narrative that links the numerical data to real-world contexts and strategic decisions.
Lastly, Data Visualization is pivotal, as it translates complex data sets into visual representations like graphs, charts, and dashboards. Visual tools make data more accessible and support the human brain's ability to recognize patterns and trends quickly.
The Dos and Don'ts in Data Analysis
While data analysis offers enormous potential, it is crucial that ethical considerations guide its application. Maintaining the privacy and security of data subjects is of utmost importance in upholding legal and moral standards. Analysts and data scientists must navigate the precarious balance between insight and intrusion, ensuring that their work is conducted with the highest ethical standards in mind.
Avoiding common follies in data analysis is crucial for accurate and reliable results. These may include making decisions based on incomplete or poorly interpreted data, falling victim to data biases, overfitting models, and drawing conclusions from correlations without establishing causality. For instance, a correlation between ice cream sales and drowning incidents does not imply causation. Analysts should be wary of such pitfalls to prevent misguided strategies.
Real-world Applications and Examples of Data Analysis
Data analysis helps segments, track performance, and optimize marketing spending. For example, a brand might analyze customer purchase histories to identify trends and produce more targeted advertising, leading to increased ROI on marketing efforts.
Data analysis has revolutionized healthcare. By scrutinizing patient data and treatment outcomes, healthcare providers can identify effective treatments and improve patient care. An example here is the use of data analysis to predict patient readmission rates.
The financial sector relies heavily on data analysis for risk management, fraud detection, and algorithmic trading. Financial institutions analyze historical trading data to predict stock performances, assist in investment strategies, and identify unusual patterns that may indicate fraudulent activities.
The Future of Data Analysis
As we forge ahead, data analysis is expected to become more sophisticated and integral to decision-making. The acceleration in data volume, variety, and velocity points towards more advanced analytics tools and methodologies in the future.
As analytics becomes more pervasive, the potential for growth and learning in the field of data analysis is immense. Businesses must be willing to adapt to these forthcoming shifts by investing in the necessary technologies, fostering a culture of data-driven decision-making, and equipping their workforce with the requisite skills. As we look toward the future, the continuous evolution of data analysis presents an exciting opportunity for professionals and students alike to expand their knowledge and skills.
Final Reminder and Blog Summary
This blog post has journeyed through the essence and efficacy of data analysis in today's business ecosystem. From descriptive to prescriptive Analysis, the data processing layers have been unwrapped, demonstrating their utility and implications in various real-world scenarios.
Encouraging a deeper dive into the world of data analysis is not only suitable for those looking to enhance their problem-solving skills training or participate in online certificate courses but also integral for any professionals aiming to excel in the modern workplace. As every sector becomes increasingly data-centric, the mastery of data analysis is no longer optional but a categorical imperative.

He is a content producer who specializes in blog content. He has a master's degree in business administration and he lives in the Netherlands.