Statistical Analysis: Key Approach for Data Interpretation and Decision Making
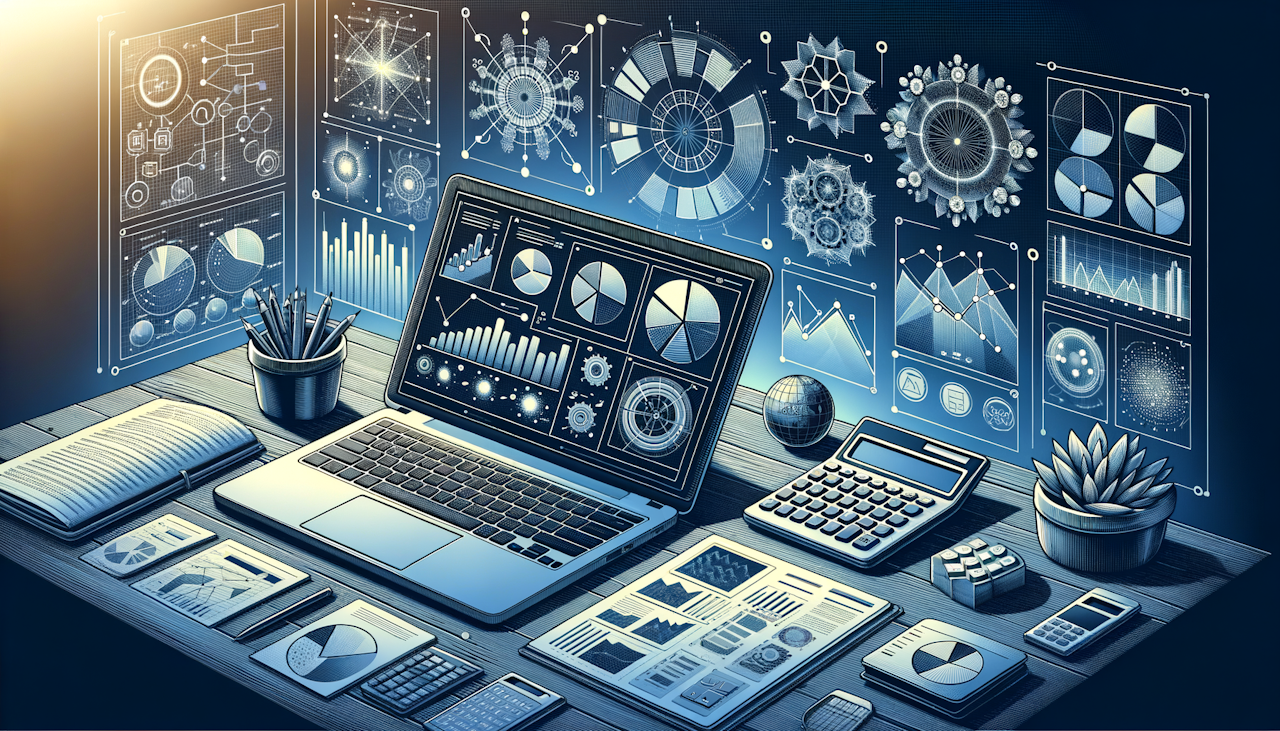
In an age of information overload, the ability to decipher the deluge of data stands as one of the most coveted skills across industries. The role of statistical analysis in interpreting this data cannot be understated. With its roots reaching deep into the scientific method, statistical analysis provides a structured approach to making sense of numbers, thereby guiding decisions. This article aims to shed light on the basics, importance, and intricacies of statistical analysis for effective data interpretation and decision-making.
Understanding the Basics of Statistical Analysis
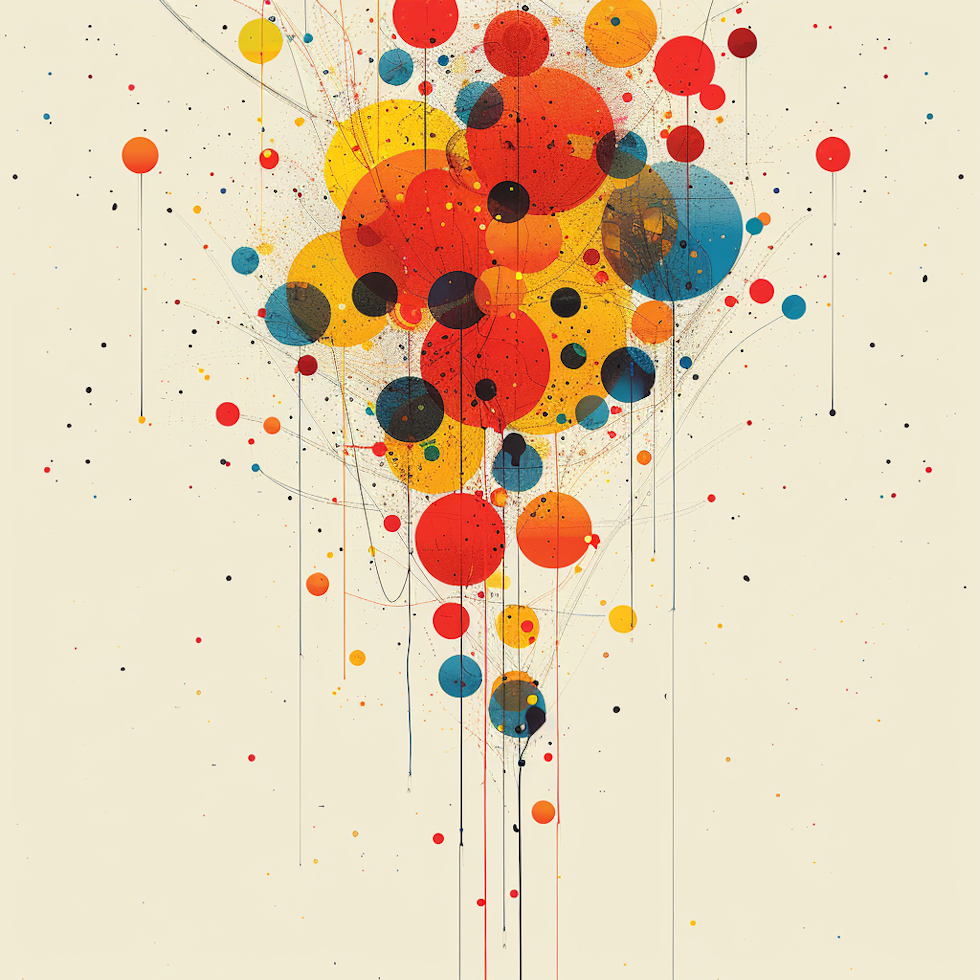
Statistical analysis, though sometimes daunting to the uninitiated, starts with grasping a few foundational concepts. The statistical population refers to a complete set of items that share at least one property of interest. A sample, by contrast, is a subset of this population, ideally representative so that one can infer conclusions back to the whole.
The variable, then, is an observable characteristic of each element in the population or sample which can vary from one entity to another. Finally, a hypothesis in statistics stands as a formal statement predicting the relationship between variables, tested through the analysis.
Definition of key terms
Statistics are broadly categorized into two types: descriptive and inferential. Descriptive statistics summarize data through figures like mean or median — they describe. Inferential statistics, however, take a leap into generalizing about a population based on a sample, making assumptions and predictions or testing a hypothesis. This dichotomy lays the foundation for the complex techniques that analysts employ to extract meaning from data.
Two primary types of statistics
In practice, a robust understanding of both descriptive and inferential statistics could be a game-changer for decision-making processes across fields. Every sector, from business to healthcare, relies on data insights to drive strategic decisions. For example, in business, statistical analyses help in customer segmentation and product optimization based on trends observed through data. In health and medicine, statistics underpin clinical trials and public health policies.
Application of statistics in different fields
Statistical analysis is no longer a tool reserved for the impassive spreadsheet - it's an arsenal utilized by a broad array of professionals. A business analyst might use A/B testing to determine the most effective marketing strategy while a medical researcher could rely on survival analysis to evaluate treatment efficacy. Such applications underscore the versatility of statistical tools in problem-solving and the value of online certificate programs in equipping professionals with these skills.
Steps Involved in Conducting Statistical Analysis
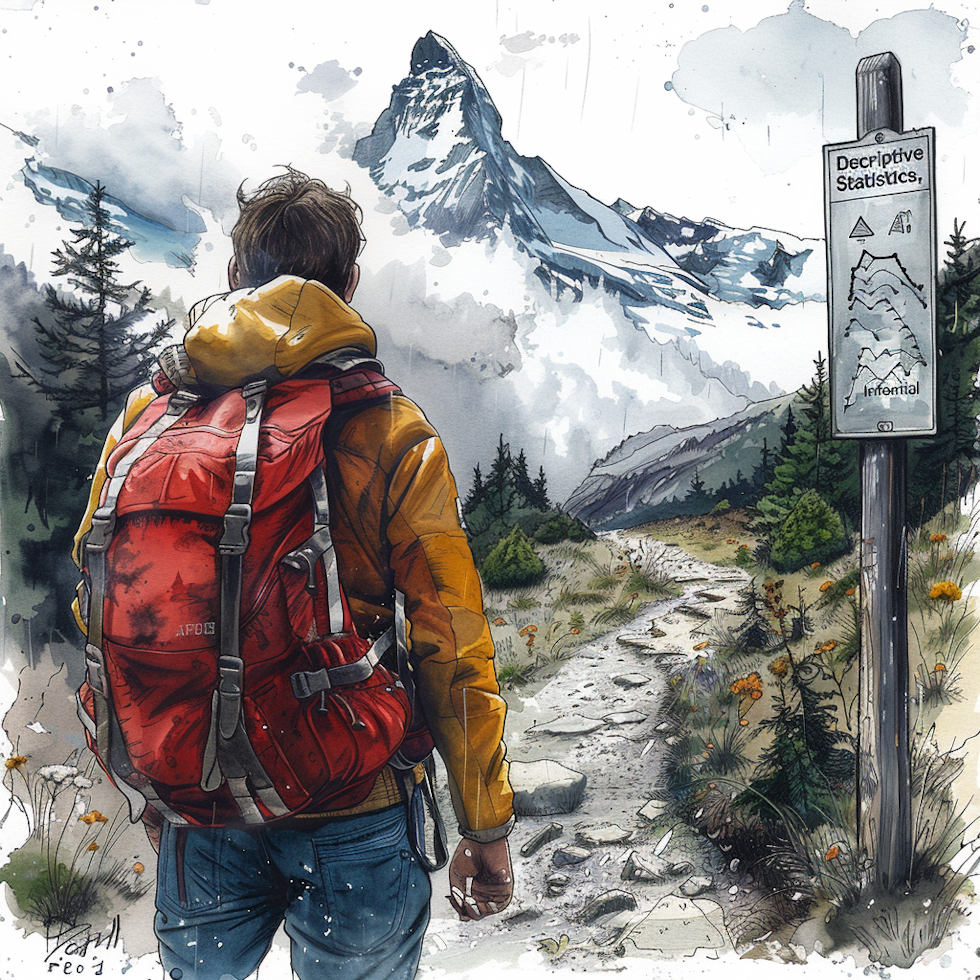
Before the numbers dance and the graphs sing, a structured approach is key. First and foremost, a clear research question or problem must be identified. This framing sets the compass for the entire analytical process, signposting what data is necessary and how one might go about obtaining it.
Identifying the research question or problem
Once the direction is set, gathering data ensues. This crucial step can be cumbersome and expensive, but its importance is paramount. An understanding of the population helps inform the methods for data collection, which could range from surveys and experiments to observational studies.
Collection of data
Inferential statistics are specifically informative here — how does one obtain a representative sample? What sampling strategies will yield the best predictive power? These questions greet the data collector with the dawn of every new dataset.
Data analysis
Data analysis itself is a meticulous venture. Cleaning data ensures its quality, free of duplicates or errors, while coding data mechanizes its interpretation. Subsequently, data must be input into analytic software or databases, translating the rawness of reality into the sleek columns of digital interfaces.
Interpretation of results
Interpretation swings back to the statistical heartland — the joys of deriving meaning. Here, spread and central tendency measures like standard deviation and mean gain their spotlight. They inform us not just of what is, but how it is distributed and in what way it may differ from our expectations.
Presentation of results
Lastly, data must be presented in a comprehensible form. Visuals such as graphs and tables literally picture the abstract, making the intricate digestible. Even the most complex findings can be communicated with elegance when draped in visual clarity.
Commonly Used Statistical Analysis Techniques
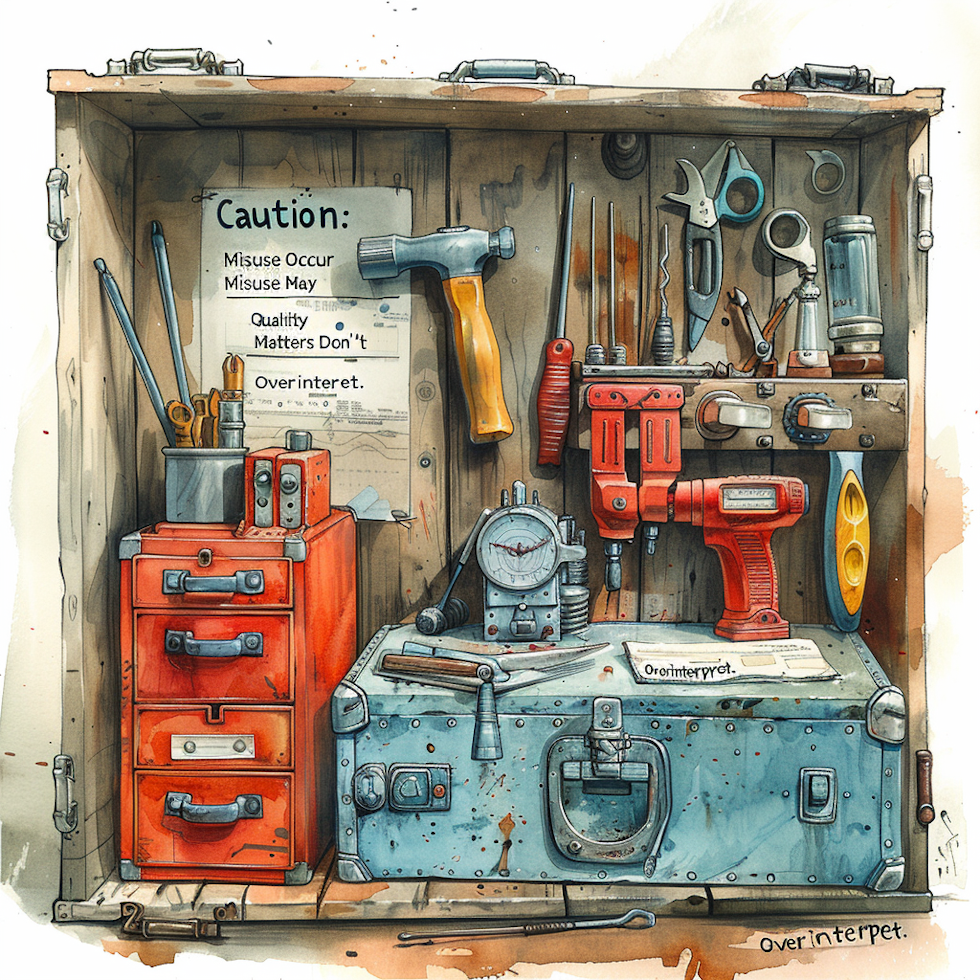
Against the backdrop of raw data, certain statistical techniques star more frequently than others. Both parametric and non-parametric statistical techniques have their place — the former assumes data follows a certain distribution like normality, the latter makes no such assumption, broadening its applicability.
Parametric and Non-parametric statistical techniques
Correlation and regression analysis offer insights into relationships between variables — does an increase in study time correlate with higher test scores? How significantly does temperature influence ice cream sales? These questions lie in the realm of correlation and regression.
Correlation and Regression Analysis
Analysis of Variance (ANOVA) allows comparison across groups. Is there a statistical difference in the average income levels of different cities? ANOVA can inform such inquiries. Similarly, the Chi-Square test helps unravel the association between categorical variables, and Factor Analysis zooms out to reveal underlying dimensions in complex datasets.
Analysis of Variance (ANOVA)
Each of these techniques, and several others, is bolstered by the power of statistical software, tools like SPSS, SAS, and R. These programs transform the laborious into the feasible. As such, a problem solving certificate that aligns with the use of statistical software enhances a professional's ability to wield these momentous technological aids.
Chi-Square test
In essence, the methodology selected pivots on the nature of the data and the questions asked. A judicious selection of technique, guided by expert judgment and a sound understanding of statistical principles, fortifies the entire analysis.
Factor Analysis
Acknowledging the robustness of statistical techniques, we concurrently confront their limitations and challenges. Incorrect or biased data collection can skew results, leading to false conclusions. Misinterpretation is another menace; a lack of understanding in the statistical domain can lead to erroneous interpretations of true findings, and an overreliance on statistical significance overlooks the importance of practical significance.
Limitations and Challenges of Statistical Analysis
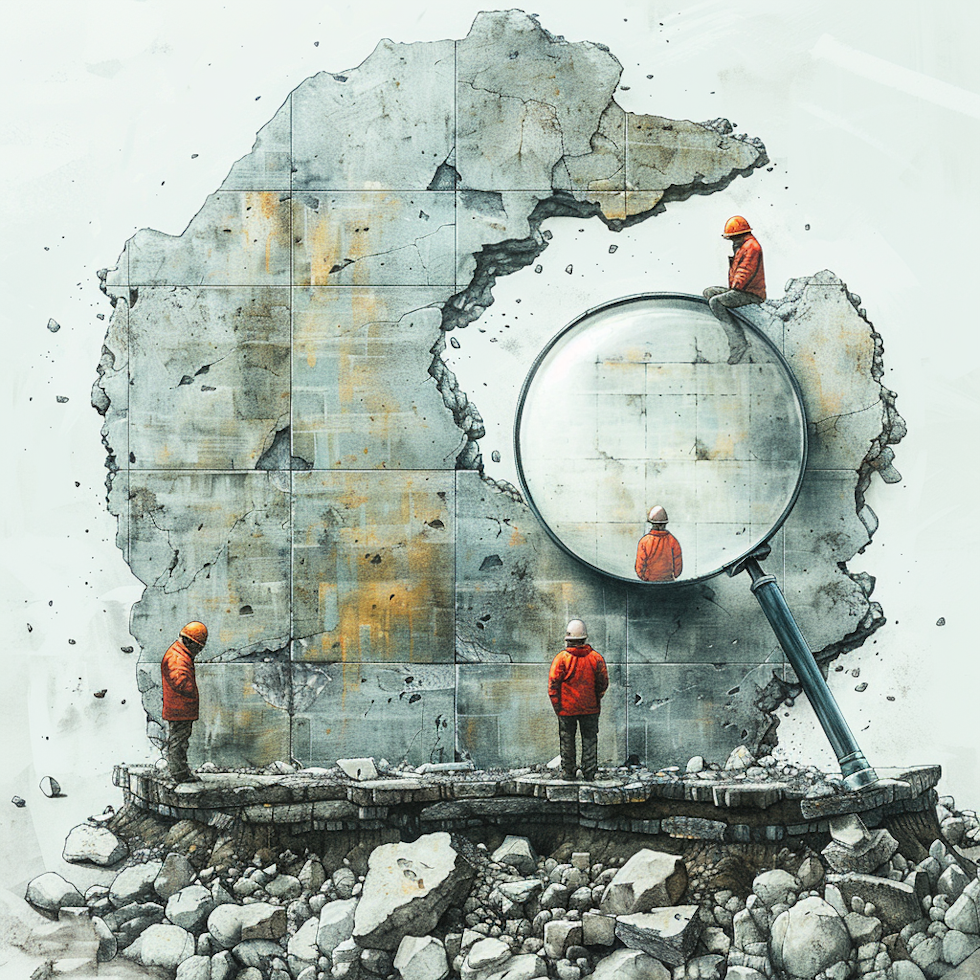
Bias in data collection is akin to building a house on a slant — the end result is distorted. Researchers must exercise caution in how data is gathered, ensuring that the process is as objective and inclusive as possible.
Incorrect or biased data collection
Misinterpretation of results is a silent foe; it can creep in unnoticed, leading well-meaning analysts astray. Precision in reading the statistical tea leaves is as important as the techniques themselves.
Misinterpretation of results
An overreliance on the p-value, the measure commonly used to determine statistical significance, can mislead. It fails to tell the complete story and may lead analysts to overlook the practical implications of their findings.
Overreliance on statistical significance
At the heart of these challenges lies a broader issue — a widespread lack of statistical literacy. As data's role in society balloons, so too must our collective comprehension of statistical principles.
Conclusion
The bedrock principles of statistical analysis undeniably augment the value of data. By utilizing statistical thinking and techniques, decision-makers across disciplines can cut through the complexity of information, extract meaningful patterns, and make informed choices. The article affirms the urging need for enhanced statistical understanding — a clarion call that can be answered by engaging with educational offerings such as online certificate programs. As our computational tools evolve and data proliferates, the strategic application of statistical analysis remains a pillar of insight — a beacon in our quest to harness the vast and untamed seas of information.

He is a content producer who specializes in blog content. He has a master's degree in business administration and he lives in the Netherlands.