Sensitivity Analysis: An Insightful Approach to Data Interpretation
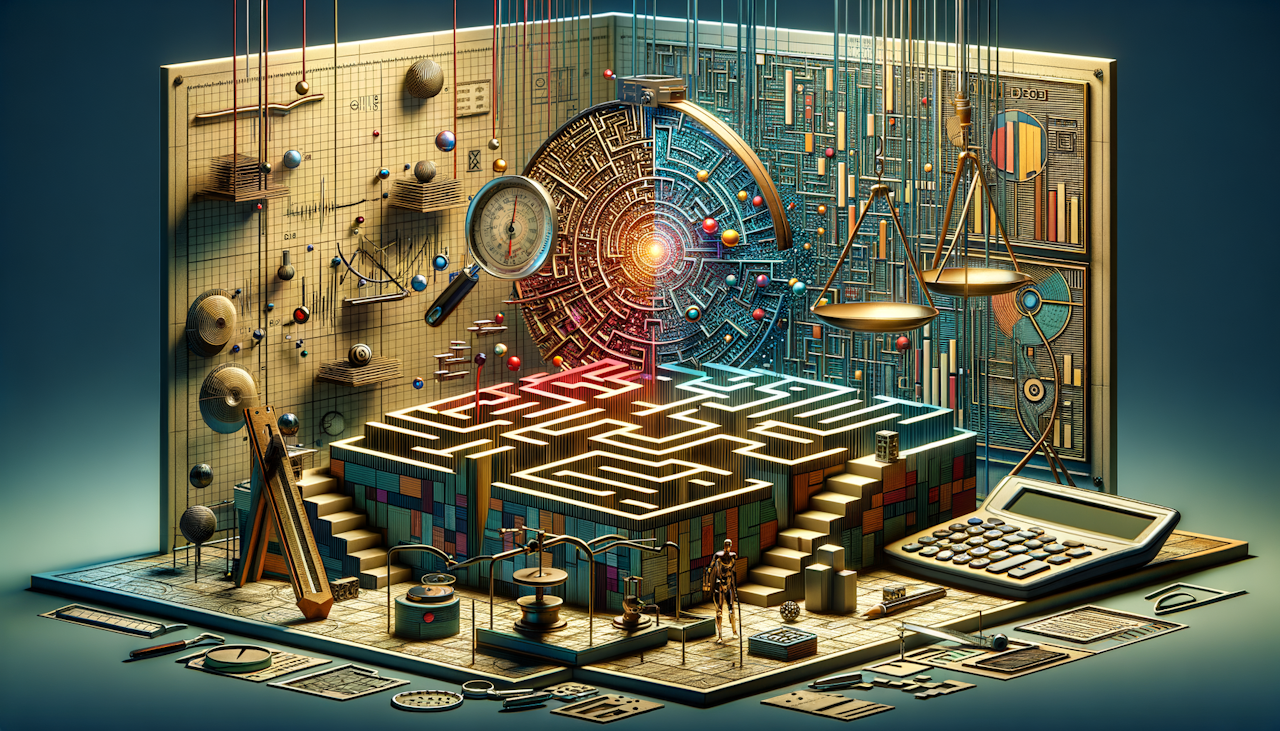
As someone who has spent years working with data across various industries, I've come to appreciate the immense value of sensitivity analysis in making informed decisions. It's a tool that has helped me navigate complex problems, assess risks, and uncover insights that would have otherwise remained hidden. In this blog post, I want to share my personal experience with sensitivity analysis and explore its theoretical framework, types and techniques, the process of performing it, its role in decision making, and potential limitations. My aim is to provide a comprehensive overview that will help you understand and apply this powerful approach in your own work.
The Essence of Sensitivity Analysis: A Theoretical Framework
At its core, sensitivity analysis is about understanding how changes in input variables affect the output of a model. It's a systematic way of exploring the relationships between different factors and quantifying their impact on the results. When I first encountered sensitivity analysis during my early days as a data analyst, I was fascinated by its ability to provide a clear picture of what really matters in a complex system.
Key Concepts in Sensitivity Analysis
To grasp the essence of sensitivity analysis, it's crucial to understand a few key concepts:
Inputs: These are the variables that can be changed or controlled in a model. They can be anything from numerical parameters to categorical variables.
Outputs: These are the results or outcomes of the model that we're interested in analyzing. They depend on the values of the input variables.
Sensitivity: This refers to how much the output changes in response to changes in the inputs. A highly sensitive variable will have a significant impact on the results, while a less sensitive one will have a smaller effect.
I remember struggling with these concepts when I first started, but as I worked on more projects and saw sensitivity analysis in action, they became clearer. It's like putting together a puzzle – once you understand how the pieces fit, the whole picture becomes much more meaningful.
Exploring Different Types and Techniques of Sensitivity Analysis
One of the things I love about sensitivity analysis is the variety of techniques available. Depending on the complexity of the model and the questions you're trying to answer, you can choose from a range of approaches. Let's take a closer look at some of the most common ones.
One-at-a-Time (OAT) Sensitivity Analysis
OAT is the simplest and most intuitive type of sensitivity analysis. As the name suggests, it involves changing one input variable at a time while keeping all others constant, and observing the effect on the output. I've found OAT to be particularly useful when I'm dealing with a small number of variables and want to get a quick sense of their relative importance.
I once used OAT to analyze the factors affecting customer churn in a telecom company. By varying each factor individually, I was able to identify the top three drivers of churn and recommend targeted retention strategies. It was a straightforward analysis, but it provided valuable insights that helped the company reduce churn and improve customer satisfaction.
Global Sensitivity Analysis (GSA)
GSA is a more advanced technique that explores the entire input space simultaneously. Instead of changing one variable at a time, GSA looks at all possible combinations of input values and assesses their joint effect on the output. This approach is particularly useful when dealing with complex models that have many interacting variables.
I recently used GSA to evaluate the robustness of a financial risk model. By simulating thousands of scenarios with different input values, I was able to identify the key drivers of risk and assess the model's sensitivity to various assumptions. The results helped the company refine its risk management strategies and make more informed investment decisions.
Local Sensitivity Analysis (LSA)
LSA focuses on the sensitivity of the output to small changes in the inputs around a specific point. It's useful when you're interested in understanding the behavior of the model in a particular region of the input space, such as the optimal solution or a critical threshold.
I applied LSA to optimize the production process in a manufacturing plant. By analyzing the sensitivity of the output quality to small variations in temperature, pressure, and other process parameters, I was able to identify the optimal operating conditions and reduce waste. The plant manager was thrilled with the results and implemented the recommendations right away.
The Process of Performing
Performing sensitivity analysis is a systematic process that involves several key steps. Over the years, I've developed a structured approach that helps me ensure the accuracy and reliability of my results. Let me walk you through the main stages of the process.
Defining the Problem and Identifying the Variables
The first step is to clearly define the problem you're trying to solve and identify the relevant input and output variables. This may seem obvious, but it's crucial to get it right. I once spent weeks analyzing a complex supply chain model only to realize that I had overlooked a critical input variable. It was a frustrating experience, but it taught me the importance of taking the time to properly define the scope of the analysis.
When identifying the variables, it's important to consider both the controllable factors (those that can be changed) and the uncontrollable ones (those that are fixed or uncertain). I usually start by brainstorming with the team and consulting with subject matter experts to ensure that I'm not missing anything important.
Developing the Model and Collecting Data
Once you have identified the variables, the next step is to develop a mathematical or computational model that represents the system you're analyzing. This could be anything from a simple spreadsheet to a complex simulation model. The key is to strike a balance between accuracy and simplicity – you want the model to be detailed enough to capture the essential features of the system, but not so complex that it becomes difficult to understand and interpret.
Collecting data is another critical step in the process. You need reliable and representative data to feed into the model and generate meaningful results. I've learned the hard way that garbage in equals garbage out – if you use poor quality data, you'll get poor quality results. That's why I always take the time to validate the data and check for errors or inconsistencies.
Running the Analysis and Interpreting the Results
With the model and data in place, it's time to run the sensitivity analysis. This involves varying the input variables according to a predefined plan and observing the effect on the output. Depending on the technique you're using (OAT, GSA, LSA), you may need to run multiple simulations or experiments to cover the entire input space.
Interpreting the results is where the real value of sensitivity analysis lies. It's not just about crunching numbers – it's about understanding what the numbers mean and how they can inform decision making. I like to use a combination of graphical and statistical tools to visualize the results and identify the most important variables. I also make sure to document my findings and communicate them clearly to the stakeholders.
The Role of Sensitivity Analysis in Decision Making
Sensitivity analysis is not just an academic exercise – it has real-world implications for decision making. I've seen firsthand how it can help organizations make better choices, manage risks, and optimize their operations. Let me share a couple of examples from my own experience.
Informing Investment Decisions
In one of my previous roles, I worked with a venture capital firm that was considering investing in a new technology startup. The startup had a promising product, but there were many uncertainties around its market potential, competitive landscape, and financial projections. I used sensitivity analysis to evaluate the robustness of the startup's business model and identify the key drivers of its success.
By varying assumptions around market size, adoption rate, pricing, and other factors, I was able to create a range of scenarios and assess the potential return on investment. The analysis revealed that the startup was highly sensitive to changes in customer acquisition costs and retention rates. Armed with this insight, the firm was able to negotiate better terms with the startup and structure the investment in a way that mitigated the risks.
Optimizing Resource Allocation
In another project, I worked with a nonprofit organization that was struggling to allocate its limited resources effectively. The organization had multiple programs and initiatives, each with its own objectives and constraints. I used sensitivity analysis to evaluate the impact of different resource allocation strategies on the organization's overall performance.
By varying the budget allocations and other parameters, I was able to identify the programs that had the greatest leverage and the ones that were most sensitive to changes in funding. The analysis also revealed opportunities for synergies and cost savings across different programs. Based on these insights, the organization was able to optimize its resource allocation and improve its impact on the community.
Limitations and Challenges of Sensitivity Analysis
While sensitivity analysis is a powerful tool, it's not without its limitations and challenges. As with any analytical technique, it's important to be aware of the potential pitfalls and take steps to mitigate them. Here are a few key issues to keep in mind.
Dealing with Uncertainty and Variability
One of the biggest challenges in sensitivity analysis is dealing with uncertainty and variability in the input variables. In many cases, you may not have precise values for the inputs, but rather ranges or distributions. This can make it difficult to determine the appropriate level of variation to use in the analysis.
I've found that using probabilistic techniques, such as Monte Carlo simulation, can be helpful in these situations. By assigning probability distributions to the input variables and running multiple simulations, you can get a sense of the range of possible outcomes and the likelihood of different scenarios.
Interpreting the Results in Context
Another challenge is interpreting the results of the sensitivity analysis in the proper context. It's easy to get caught up in the numbers and lose sight of the bigger picture. I always try to step back and ask myself what the results really mean for the problem at hand.
For example, just because a variable is highly sensitive doesn't necessarily mean that it's the most important one to focus on. There may be other factors, such as cost, feasibility, or strategic considerations, that need to be taken into account. It's important to have a holistic view and consider the results in the context of the overall decision-making process.
Communicating the Results Effectively
Finally, communicating the results of the sensitivity analysis to stakeholders can be a challenge. Not everyone is comfortable with technical jargon or complex graphs, so it's important to find ways to present the insights in a clear and accessible way.
I've found that using visual aids, such as charts and infographics, can be very effective in conveying the key messages. I also try to use plain language and avoid unnecessary details. The goal is to help the decision makers understand the implications of the analysis and make informed choices based on the results.
Conclusion
Sensitivity analysis is a valuable tool for anyone working with data and models. It provides a structured way to explore the relationships between variables, assess risks, and make informed decisions. By understanding the theoretical framework, techniques, and process of sensitivity analysis, you can apply it effectively in your own work.
Of course, mastering sensitivity analysis takes time and practice. It's not a one-size-fits-all solution, and there will always be challenges and limitations to navigate. But with persistence and a willingness to learn, you can develop the skills and intuition needed to use sensitivity analysis to its full potential.
If you're interested in learning more about sensitivity analysis and other data-driven techniques, there are many resources available. Online courses, tutorials, and case studies can provide valuable insights and hands-on experience. And of course, nothing beats working on real-world projects and learning from your own successes and failures.
So go ahead and give sensitivity analysis a try. Embrace the power of data and use it to make better decisions. And most importantly, have fun exploring the fascinating world of data analysis!

He is a content producer who specializes in blog content. He has a master's degree in business administration and he lives in the Netherlands.