Design of Experiments (DOE): A Comprehensive Overview on Its Meaning and Usage
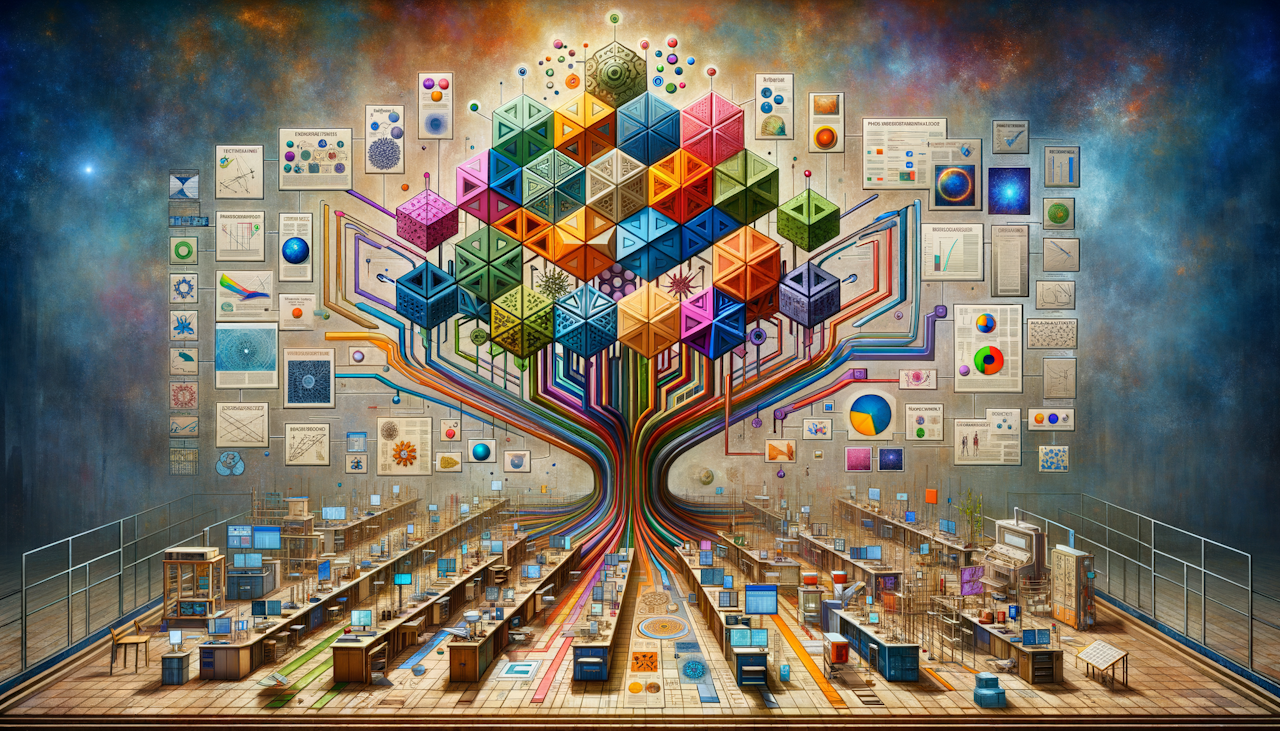
The methodological framework known as Design of Experiments (DOE) is transformative in various disciplines, allowing researchers and practitioners the ability to plan, conduct, analyze, and interpret controlled tests to evaluate the factors that may influence a particular outcome. Historically grounded in agricultural and scientific research, DOE has burgeoned into an indispensable tool across a myriad of industries, including manufacturing, healthcare, and marketing. This article aims to elucidate the concepts and applications of Design of Experiments, providing a panoramic as well as an in-depth view of its various principles, types, statistical underpinnings, and practical applications.
Introduction to Design of Experiments (DOE)
Definition and basics of Design of Experiments
Design of Experiments, at its core, is a structured methodological approach used to determine the relationship between factors affecting a process and the output of that process. It encompasses a vast array of strategies for testing hypotheses concerning the factors that could influence a particular variable of interest. The primary aim is not only to affirm the effect of these factors but also to quantify the extent to which they influence results, thus providing a scientific basis for decision-making.
Importance and relevance of DOE
In the contemporary landscape of problem-solving, the significance of DOE is multifaceted. It facilitates a systematic approach to experimentation that is both efficient and economical, reducing the number of trials needed to gather meaningful data. For instance, industries seeking to enhance their product quality can rely on DOE to guide them in identifying significant variables and their optimal settings. Moreover, DOE is an integral component of an effective problem solving techniques course or online certificate courses, as it equips students and professionals with the analytical skills necessary to tackle complex challenges.
Brief history and development of DOE
The foundations of Design of Experiments can be traced back to the early 20th century with the pioneering work of Ronald A. Fisher in the realm of agricultural research. His seminal contributions laid the groundwork for modern DOE, particularly in the context of controlling variation and maximizing information gain. As statistical methods have evolved, DOE has continued to grow in sophistication, integrating advances in computation and information technology to broaden its applicability across various fields.
Key Principles of Design of Experiments (DOE)
Randomization
What is randomization in DOE
Randomization is the backbone of a rigorous experimental design as it mitigates the effects of uncontrolled variables, or confounding factors, ensuring that the treatment groups are comparable. By assigning experimental units to treatment conditions randomly, researchers can confidently attribute differences in outcomes to the factors under study rather than to extraneous variables.
Benefits and significance of randomization
The paramount importance of randomization lies in its capacity to elevate the internal validity of an experiment. It reduces bias and ensures an unbiased estimate of the treatment effect, thus bolstering the credibility of the experimental results. This key principle is an assurance that the experiment's findings can be generalized beyond the study parameters.
Examples illustrating randomization
Consider a clinical trial for a new pharmaceutical product. If participants are randomized into the control and treatment groups, any difference observed in outcomes can be attributed to the drug's effectiveness rather than patient characteristics. This methodological rigor is what makes randomization indispensable in DOE.
Replication
Understanding replication in the context of DOE
Replication is the repeated application of certain conditions within an experiment to ensure that results are not anomalies. It adds precision to the experiment by allowing variance estimation, which is crucial for assessing the reliability of the findings.
Its role and importance in DOE
The role of replication in DOE cannot be overstressed. It affords the experimenter the ability to discern true effects from random errors, thus confirming the consistency of experimental results. When used judiciously, replication improves the power of the experiment, enabling more definitive conclusions.
Applicable examples of replication
For instance, verifying the strength of a newly engineered material could involve subjecting multiple samples to stress tests under identical conditions. The consistency in the failure threshold across these samples would indicate the reliability of the material's design as determined through replication.
Blocking
Concept of blocking in DOE
Blocking is a technique used to account for variability among experimental units that cannot be eliminated. By grouping similar experimental units together and carrying out the same experimental conditions within these blocks, one can control the variables leading to variability within each group, thus reducing overall experimental error.
Advantages of using blocking in DOE
Employing blocking in an experiment minimizes the confounding effects of variables that are known but not directly of interest to the study. This approach increases the precision of the estimates of main effects and interactions by isolating the block-to-block variability from the treatment effects. It is particularly useful when one wants to account for heterogeneity among subjects or any other nuisance variable.
Real-world blocking examples
In an agricultural study, fields could be blocked by soil type before the application of different fertilization regimes. This ensures that variation due to soil composition does not skew the results, thus giving a clearer understanding of the fertilizers' effectiveness.
Categories of Design of Experiments (DOE)
Full-factorial Design
Overview and basic understanding
Full-factorial design involves the investigation of every possible combination of factors and their levels. Each treatment combination is applied to separate units in an experiment, making it a comprehensive approach that allows for the assessment of both main effects and interactions between factors.
Strengths and limitations
While the full-factorial design provides a wealth of information about the factors in question, it can be resource-intensive and impractical when dealing with a large number of factors. However, if resources allow, this type of design delivers the most exhaustive data on the effects and possible interactions.
Use case example of full-factorial design
A manufacturer could use a full-factorial design to understand the impact of temperature and pressure on the strength of a welded joint. By varying these factors systematically across all levels, the manufacturer could determine the optimal conditions for the welding process that ensure joint strength.
Fractional-factorial Design
Definition and understanding of the concept
Fractional-factorial design is an efficient variation of the full-factorial design that investigates only a subset of the possible combinations of factor levels. By strategically selecting a fraction of the full design, researchers can still gather information on the most crucial factors influencing an outcome while significantly reducing the number of experiments.
Advantages and drawbacks
This design's main advantage is its economy in terms of time and resources. Nonetheless, this comes with the potential drawback of confounding, which is when two or more factor effects cannot be separated due to the design's reduced size. Researchers must balance the need for information with the constraints of their experimental budget.
Case study demonstrating the use of fractional-factorial design
A tech company wanting to assess the design features impacting user engagement might employ a fractional-factorial design to investigate a manageable subset of combinations rather than exhaust every potential variation, thereby identifying critical design elements efficiently.
Response Surface Design
Explaining Response Surface Design
Response Surface Design (RSD) is focused on modeling the relationship between a response and a set of quantitative variables. It is particularly useful when the goal is optimization, as RSD can identify the levels of factors that lead to the best possible outcome.
Pros and cons of using Response Surface Design
RSD exhibits potency in exploring complex, nonlinear relationships and can be pivotal in honing in on optimal conditions. However, constructing a suitable response surface model often requires a more significant number of experimental runs compared to simpler designs, and interpreting the resulting model may necessitate a substantial level of statistical expertise.
Applicable example of response surface design
An example might involve a food scientist employing RSD to optimize a recipe for flavor and texture by manipulating ingredient ratios and cooking times. By analyzing the response surface, the scientist can pinpoint the exact conditions that yield the best culinary result.
Statistical Analysis in Design of Experiments (DOE)
Role of statistical analysis in DOE
Statistical analysis is fundamental in DOE, as it transforms experimental data into meaningful insights. Here, analytic techniques are employed to discern patterns, test hypotheses, and derive conclusions that can withstand scrutiny within the scientific community.
Various statistical techniques applied in DOE
The array of statistical methods applied within DOE is expansive, encompassing t-tests, ANOVA, regression analysis, and multivariate techniques, among others. Selecting the appropriate method hinges on the complexity of the data structure and the goals of the research.
Examples demonstrating the importance of statistical analysis in DOE
For instance, a multinational corporation might conduct a series of experiments to improve a product feature. Utilizing ANOVA, researchers can determine whether differences in quality metrics across various production sites are statistically significant, guiding quality improvement efforts.
Practical Applications of Design of Experiments (DOE)
DOE in product and process development
DOE plays a critical role in new product development and process refinement. By assessing the impact of variable changes, designers and engineers can develop superior products and streamline processes for improved performance and cost-efficiency.
The role of DOE in industrial manufacturing
In the context of industrial manufacturing, DOE is an invaluable asset. It assists in identifying key process factors and optimizing manufacturing conditions, resulting in enhanced quality control and reduced production costs—a boon for competitiveness in the market.
Case studies showing the impact of DOE in various fields
Case studies from the pharmaceutical industry to aerospace engineering demonstrate DOE's vital contributions. Whether optimizing drug formulations or adjusting flight parameters, DOE's applications are incredibly diverse and contribute significantly to scientific and technological advancements.
Conclusion: Future Trends and Developments in Design of Experiments (DOE)
Current trends in DOE
The recent trends in DOE point towards the integration of more advanced computational techniques, like machine learning algorithms, to handle complex, high-dimensional data sets and to refine predictive models for experimental outcomes.
Future outlook and potential advancements in DOE
Looking ahead, we may witness the further development of real-time analytics in DOE, enabling even more dynamic exploration of experiment spaces, perhaps leading to adaptive experimentation that could revolutionize fields as disparate as genomics and supply chain management.
Final thoughts and recommendations for further studies in DOE
As the landscape of DOE progresses, so does the need for educational pursuits such as online certificate courses in this area, fostering a new generation of adept experimenters equipped with equipped with the latest tools and techniques. Investigators are encouraged to undertake further studies in DOE to harness its full potential—a testament to the enduring relevance and flexibility of this methodological powerhouse.

He is a content producer who specializes in blog content. He has a master's degree in business administration and he lives in the Netherlands.